Uncovering DeepSeek's Staggering 500% Profit Margin - Insights Revealed
Uncover the staggering 500% profit margin of DeepSeek's AI inference services. Discover how their innovative approach to infrastructure and pricing disrupts the industry. Optimize your AI models with these insightful learnings.
22 tháng 4, 2025

DeepSeek's innovative approach to inference has enabled them to achieve an astounding 500% profit margin, showcasing their ability to deliver high-performance AI services at a fraction of the cost. This blog post delves into the insights behind their remarkable success, offering valuable lessons for businesses seeking to optimize their AI infrastructure and pricing strategies.
How DeepSeek is Achieving a 500% Profit Margin
Optimized Inference System with High-Performance GPUs
Efficient Load Balancing and Resource Utilization
Impressive Capacity and Processing Capabilities
Analyzing the Revenue and Profit Potential
Comparison to Industry Pricing and Profitability
Conclusion
How DeepSeek is Achieving a 500% Profit Margin
How DeepSeek is Achieving a 500% Profit Margin
DeepSeek's inference system for their R1 and V3 models is highly optimized, allowing them to achieve an astounding 500% profit margin. They are using H100 GPUs for inference, maintaining the same precision as the training process. To efficiently manage their infrastructure, DeepSeek has implemented a smart load-balancing mechanism, deploying inference services across all nodes during peak daytime hours, while utilizing the extra capacity for research and training purposes during off-peak hours.
Over the last 24 hours, DeepSeek had a peak of 278 nodes, with an average occupancy of 226 nodes, processing a total of 600 billion input tokens, of which half hit their KV cache, resulting in 168 billion output tokens generated at a speed of 20-22 tokens per second. This massive scale of inference operations, combined with their ownership of the GPU infrastructure, allows DeepSeek to achieve an estimated daily revenue of around $500,000, with an actual profit margin of approximately 85%.
The key factors contributing to DeepSeek's exceptional profitability are their innovative infrastructure optimization, strategic pricing, and the inherent advantages of owning their GPU resources. This level of efficiency and profitability sets DeepSeek apart from other foundation model API providers, who may be struggling to achieve sustainable business models.
Optimized Inference System with High-Performance GPUs
Optimized Inference System with High-Performance GPUs
DeepSee's inference system for their R1 and V3 models is highly optimized and leverages powerful H100 GPUs. They ensure that the inference is performed with the same precision as the training, maintaining the model's accuracy.
To efficiently manage the inference workload, DeepSee has implemented a smart load-balancing mechanism. During peak daytime hours, they deploy inference services across all available nodes to handle the high traffic. However, during off-peak nighttime hours, they utilize the extra capacity for research and training purposes.
The inference system is designed to be highly scalable, with a total of 278 nodes at peak and an average occupancy of 226 nodes. This translates to approximately 2,224 H100 GPUs being used for inference, costing around $887,000 per day.
The system's optimization is further evident in the efficient utilization of the key-value cache, where half of the 600 billion input tokens processed in the last 24 hours were served from the cache, reducing the computational load.
Despite the significant inference costs, DeepSee's revenue numbers are staggering. They estimate a daily revenue of around $500,000, which would result in a profit margin of approximately 500% compared to the inference costs. This is primarily due to their pricing strategy, where they have significantly reduced the prices for their V3 and R1 models, especially during off-peak hours.
The combination of high-performance GPUs, efficient load balancing, and strategic pricing has allowed DeepSee to achieve remarkable profitability in their inference operations, showcasing their innovative approach to serving their models at scale.
Efficient Load Balancing and Resource Utilization
Efficient Load Balancing and Resource Utilization
DeepSee's inference system is designed to efficiently manage its resources and optimize its operations. During peak daytime hours, the system deploys inference services across all available nodes to handle the high load. However, during off-peak nighttime hours, the system utilizes the extra capacity for research and training purposes, ensuring that the resources are fully utilized.
The system's capacity is well-optimized, as evidenced by the small difference between the peak and average occupancy. Over the last 24 hours, the system had a peak of 278 nodes and an average of 226 nodes, indicating a highly efficient load balancing mechanism.
Furthermore, the system leverages a smart caching mechanism, where half of the 600 billion input tokens processed in the last 24 hours were served from the key-value (KV) cache, resulting in improved performance and reduced computational load.
The system's efficient resource utilization and optimization strategies have enabled DeepSee to achieve remarkable profitability. Despite significantly lowering their pricing for the V3 and R1 models, the company is still able to maintain a staggering 500% profit margin on their inference services. This highlights the innovative approaches DeepSee has implemented to drive down costs and maximize revenue.
Impressive Capacity and Processing Capabilities
Impressive Capacity and Processing Capabilities
DeepSee's online inference system is powered by H100 GPUs, ensuring consistent performance with the training process. They have implemented a smart load-balancing mechanism that deploys inference services across all nodes during peak daytime hours, while utilizing the extra capacity for research and training purposes during off-peak hours.
Over the last 24 hours, DeepSee had a total of 278 nodes at peak, with an average occupancy of 226 nodes. This efficient utilization of resources translates to approximately 2,224 H100 GPUs, costing around $887,000 per day for inference.
Despite these costs, DeepSee's impressive processing capabilities are truly remarkable. They have processed a staggering 600 billion input tokens, with half of them hitting the KV cache, resulting in 168 billion output tokens generated at a speed of 20-22 tokens per second.
When considering the revenue generated from these processing capabilities, the numbers become even more astounding. DeepSee's daily revenue is estimated to be around $500,000, which would amount to a profit margin of approximately 500% compared to the inference costs. Even when accounting for the fact that they are only currently charging for API usage and not the web and app usage, the actual revenue and profit margins are still substantially higher than the industry standard.
This level of efficiency and profitability showcases the innovative approach DeepSee has taken in optimizing their inference system and pricing strategy, setting a new benchmark for the industry.
Analyzing the Revenue and Profit Potential
Analyzing the Revenue and Profit Potential
The key highlights from the provided information are:
- DeepSee is offering their AI models (R1 and V3) at significantly discounted prices, with up to 50% and 75% off-peak discounts.
- Despite the low pricing, DeepSee is able to achieve an estimated profit margin of around 500% on their inference services.
- This is achieved through efficient infrastructure utilization, with the same GPU clusters used for both training and inference.
- During peak hours, the inference services are prioritized, while the spare capacity is used for research and model training during off-peak hours.
- DeepSee's inference cost is estimated to be around $887,000 per day, processing 600 billion input tokens and generating 168 billion output tokens.
- The theoretical daily revenue from this inference workload is estimated to be around $0.5 million, resulting in the 500% profit margin.
- Even after accounting for the fact that only the API usage is currently monetized, and the V3 pricing is significantly lower than R1, DeepSee's actual profit margins are still estimated to be around 85%.
- This level of profitability and efficiency in AI inference operations is seen as a significant innovation by DeepSee, and it raises questions about the pricing and profitability models of other AI service providers.
Comparison to Industry Pricing and Profitability
Comparison to Industry Pricing and Profitability
The revenue numbers and profit margins revealed by DeepSee for their R1 and V3 models are truly staggering, especially when compared to the pricing and profitability of other foundation model API providers.
According to the information provided, DeepSee's inference costs are around $887,000 per day, which they are able to turn into a theoretical daily revenue of around $500,000 - a profit margin of approximately 500%. This is in stark contrast to other providers, such as OpenAI, who are reportedly losing money on their $200 packages.
The key factors contributing to DeepSee's impressive profitability include:
- Aggressive Pricing: DeepSee has slashed their prices by 50% for V3 and 75% for R1 during off-peak hours, making their models significantly more affordable than competitors.
- Efficient Infrastructure: DeepSee is leveraging their own H100 GPUs for inference, which likely reduces their costs compared to relying on third-party cloud providers.
- Optimized Utilization: DeepSee has implemented a smart load-balancing mechanism to maximize the utilization of their inference infrastructure, using spare capacity for research and training during off-peak hours.
- Caching Optimizations: Half of the 600 billion input tokens processed by DeepSee are served from their KV cache, further reducing the computational load and costs.
These factors, combined with DeepSee's ownership of the underlying infrastructure, have enabled them to achieve an estimated profit margin of around 85% - a level of profitability that is truly remarkable in the industry.
The implications of these findings are clear: DeepSee's approach to pricing, infrastructure, and optimization has the potential to disrupt the foundation model API market, forcing other providers to re-evaluate their own strategies and pricing models.
Conclusion
Conclusion
The key takeaways from the provided information are:
- DeepSpeech R1 and V3 models are being served using H100 GPUs with 8-bit precision, optimizing for cost and performance.
- They have a smart load balancing mechanism, using spare capacity at night for research and training.
- Their inference system can process up to 600 billion input tokens per day, with 20-22 tokens per second generation speed.
- The estimated daily revenue from the API usage alone is around $500,000, with a profit margin of around 500% of the inference cost.
- Even considering the discounted pricing and only monetizing a subset of services, their actual profit margins are estimated to be around 85%.
- This level of profitability and efficiency raises questions about the pricing and business models of other foundation model API providers.
Câu hỏi thường gặp
Câu hỏi thường gặp
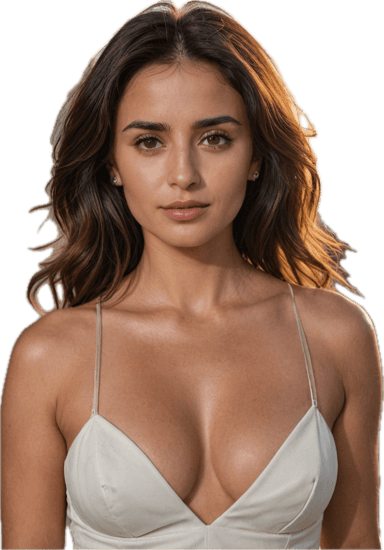
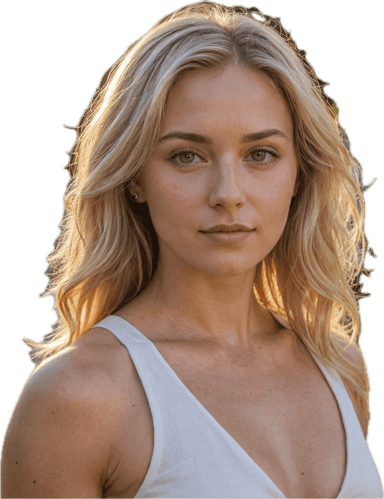