Unleash the Power of NVIDIA's Real-Time Text-to-Video AI
Unleash the power of NVIDIA's real-time text-to-video AI. Discover the latest breakthroughs in text-to-video generation, including 12x faster runtime, identity preservation, and advanced relighting capabilities. Explore the potential of these cutting-edge AI models for your creative projects.
24 de fevereiro de 2025
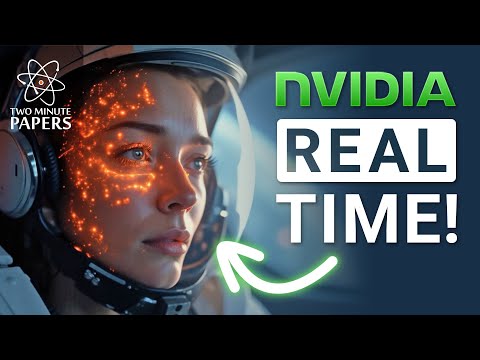
Discover the incredible advancements in AI-powered video generation that are revolutionizing content creation. Explore the latest breakthroughs, including 12x faster video generation, identity-preserving subject-to-video, and advanced video relighting capabilities. This blog post delves into the cutting-edge technologies that are transforming the way we create and consume video content.
Blazing-Fast Text-to-Video Generation: 12x Speedup!
Combining Text-to-Image and Image-to-Video for Efficiency
Preserving Identities in Subject-to-Video Generation
Relighting Videos without Changing the Content
High-Quality Video Generation with Longer Wait Times
Conclusion
Blazing-Fast Text-to-Video Generation: 12x Speedup!
Blazing-Fast Text-to-Video Generation: 12x Speedup!
This new text-to-video generation system is truly remarkable, boasting a 12x speedup compared to previous approaches. Instead of generating one-minute videos in one minute, this technique can create video footage in real-time, generating one second of video within one second.
The key innovation is a two-step process: first, it generates an image from the text prompt, and then it animates that image to create the final video. This approach allows for much faster generation times, as the system only needs to create a single video from the pre-generated image, rather than generating the entire video from scratch.
Additionally, the system employs a sparsification step, which cuts a few corners to further optimize the process and achieve near-real-time performance. Remarkably, this can be done on a single consumer-grade graphics card, making it accessible to a wider audience.
The system's capabilities are truly impressive, with the ability to create a diverse range of video content from simple text prompts. While the dataset used for training may be biased towards human-centric and cinematic content, the underlying concept is highly promising, and future models can be trained on more balanced datasets to address this limitation.
Combining Text-to-Image and Image-to-Video for Efficiency
Combining Text-to-Image and Image-to-Video for Efficiency
The key idea behind this approach is to leverage the speed and efficiency of text-to-image AI systems to generate an initial image, and then use that as input to a text-to-video AI system. This two-step process allows for faster video generation compared to directly generating the video from text alone.
The text-to-image step provides a starting point that the text-to-video model can then animate and expand upon. By avoiding the need to generate the video from scratch, the overall process becomes significantly faster, approaching real-time speeds. This is particularly useful when experimenting with different text prompts, as you can quickly generate and evaluate the resulting videos without having to wait for lengthy generation times.
Additionally, the text-to-image step helps ensure that the generated video content aligns with the desired prompt, as the model has already produced an image that matches the text input. This increases the likelihood that the final video will be satisfactory, without the need to try numerous text prompts until a suitable one is found.
Overall, this combined approach leverages the strengths of both text-to-image and image-to-video AI systems to create an efficient and effective text-to-video generation pipeline.
Preserving Identities in Subject-to-Video Generation
Preserving Identities in Subject-to-Video Generation
The new system called Phantom is capable of generating videos not just from text, but from a given subject such as a person, place, or object. The key advantage of this system is that it can preserve the identities of the subjects across the generated video frames. This is an important feature, especially for applications like creating comics or animations with a consistent central character.
While the visual quality of the Phantom system may be slightly lower compared to the text-to-video models, the ability to maintain the same identities throughout the video is a significant improvement. This allows for more coherent and recognizable content, which can be particularly useful in creative and storytelling applications.
The research paper on the Phantom system is available for further exploration, providing an opportunity for Fellow Scholars to dive deeper into this innovative approach to subject-to-video generation that preserves the integrity of the featured entities.
Relighting Videos without Changing the Content
Relighting Videos without Changing the Content
This new tool allows for the relighting of input videos without significantly altering the content. If you want to make your video look more dramatic, or place your cat in a cyberpunk world, this tool makes it easy to do so. The relighting process preserves the original video's identity and content, while enhancing the visual presentation according to your preferences. This capability provides creators with a powerful tool to refine and stylize their video content without the need for extensive editing or refilming.
High-Quality Video Generation with Longer Wait Times
High-Quality Video Generation with Longer Wait Times
Step Video is a text-to-video generation system that prioritizes visual quality over generation speed. While it may take longer to produce a video compared to the 12x real-time approach discussed earlier, Step Video promises higher-fidelity results.
The key trade-off is that users who are willing to wait a bit longer can obtain videos with more detailed and realistic visuals. This could be particularly useful for applications where the final output quality is more important than the generation time, such as in professional video production or high-end content creation.
Step Video likely employs more computationally intensive techniques to achieve its enhanced visual quality, potentially involving advanced neural network architectures, more comprehensive training datasets, or more sophisticated rendering algorithms. The increased processing time allows the system to generate videos with greater visual coherence, more natural movements, and a higher level of photorealism.
While the 12x real-time approach discussed earlier may be more suitable for rapid prototyping or quick content generation, Step Video caters to users who prioritize visual excellence over immediate results. The choice between the two systems will depend on the specific needs and requirements of the user or application.
Conclusion
Conclusion
The rapid advancements in text-to-video AI systems are truly remarkable. The ability to generate high-quality, real-time video clips from simple text prompts is a game-changer. The techniques discussed, such as using text-to-image AI as an intermediate step and leveraging sparsification, have enabled impressive performance improvements. While the current models may have some limitations, the potential for further refinement and expansion is exciting. The emergence of systems like Phantom, which can preserve identities in generated videos, and tools for video relighting, showcase the breadth of innovation in this field. The sheer pace of progress, with multiple cutting-edge models released in quick succession, is a testament to the rapid evolution of open science and open-source initiatives. As Fellow Scholars, we can eagerly anticipate the future applications and possibilities that these advancements in text-to-video AI will unlock.
Perguntas frequentes
Perguntas frequentes