De kracht van reinforcement learning ontgrendelen: inzichten in Q* en het potentieel van AI
Ontdek de mysteries achter de kracht van Reinforcement Learning. Ontdek hoe Q* en de doorbraken van AI de probleemoplossing kunnen revolutioneren en nieuwe grenzen kunnen openen. Duik in de inzichten die de toekomst van kunstmatige intelligentie kunnen vormgeven.
16 februari 2025
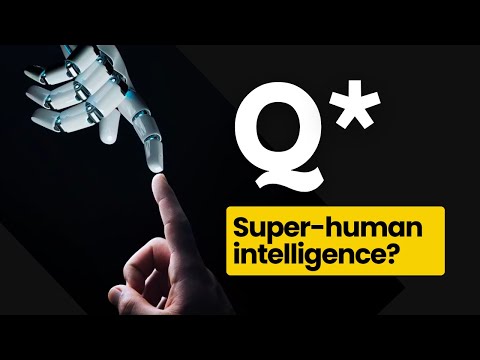
Ontgrendel de kracht van reinforcement learning en ontdek hoe het de toekomst van AI kan revolutioneren. Deze blogpost gaat in op de kernconcepten van reinforcement learning en laat zien hoe het AI-systemen in staat heeft gesteld om menselijk prestatieniveau te overtreffen in complexe taken. Verken de potentiële toepassingen van deze baanbrekende technologie en krijg inzichten die de toekomst van kunstmatige intelligentie kunnen vormgeven.
Reinforcement Learning: Een baanbrekende aanpak voor AI
De belangrijkste componenten van Reinforcement Learning
Baanbrekende voorbeelden van Reinforcement Learning
Het ontsluiten van het potentieel van grote taalmodellen met Reinforcement Learning
Conclusie
Reinforcement Learning: Een baanbrekende aanpak voor AI
Reinforcement Learning: Een baanbrekende aanpak voor AI
Reinforcement learning is a powerful machine learning framework that allows AI systems to learn and improve through trial-and-error interactions with their environment. Unlike supervised learning, which relies on labeled data, reinforcement learning agents learn by receiving rewards or penalties for their actions, gradually developing an optimal strategy to maximize future rewards.
The key components of a reinforcement learning system include:
- Agent: The AI system that interacts with the environment and takes actions.
- Environment: The setting in which the agent operates, such as a video game, simulation, or real-world scenario.
- Actions: The choices the agent can make within the environment.
- Rewards/Penalties: The feedback signals the agent receives based on the outcomes of its actions.
- Policy Network: The decision-making engine that determines the agent's actions based on the current state.
- Value Network: The component that estimates the long-term value of each possible action.
Through repeated interactions and feedback, the agent's policy network and value network reinforce each other, allowing the system to discover novel strategies and solutions that may not be obvious to human experts. This is exemplified by the achievements of DeepMind's AlphaGo, which not only surpassed human players in the game of Go but also developed unconventional moves that surprised the research team.
The potential of reinforcement learning to unlock new levels of AI capabilities is particularly exciting for large language models (LLMs) like GPT. By incorporating reinforcement learning techniques, LLMs could potentially improve their reasoning, logic, and problem-solving abilities, exploring a wider range of solutions and strategies. This could lead to breakthroughs in areas such as natural language understanding, task completion, and even creative problem-solving.
While the specifics of OpenAI's "QAR" breakthrough remain uncertain, the principles of reinforcement learning offer a promising path forward for advancing the state of the art in artificial intelligence. As the field continues to evolve, the ability of AI systems to learn and discover novel solutions through self-play and feedback-driven optimization could have far-reaching implications for the future of technology and human-AI collaboration.
De belangrijkste componenten van Reinforcement Learning
De belangrijkste componenten van Reinforcement Learning
Reinforcement learning is a machine learning framework that allows AI systems to learn from their own trials and errors. It typically consists of a few key components:
-
Agent: The agent is the AI system that interacts with the environment and takes actions.
-
Environment: The environment is the setting in which the agent operates, such as a video game, simulation, or real-world scenario.
-
Actions: The agent can take various actions within the environment, such as moving a character in a game or making a move in a strategy game.
-
State: The state represents the current condition of the environment, which the agent can observe and use to inform its decisions.
-
Rewards: The agent receives positive or negative rewards based on the outcomes of its actions, providing feedback on whether the actions were beneficial or not.
-
Policy Network: The policy network is the decision-making engine that determines which actions the agent should take in a given state to maximize the total future rewards.
-
Value Network: The value network estimates the total future rewards that the agent can expect to receive by taking a particular action in a given state.
The agent learns by repeatedly interacting with the environment, taking actions, receiving rewards, and updating its policy and value networks. Over time, the agent develops an optimal strategy for maximizing its total future rewards, which can lead to superhuman performance in certain tasks, as demonstrated by systems like AlphaGo.
Baanbrekende voorbeelden van Reinforcement Learning
Baanbrekende voorbeelden van Reinforcement Learning
Reinforcement learning has enabled AI systems to achieve superhuman performance in various tasks, showcasing the immense potential of this approach. Let's explore a few groundbreaking examples:
Breakout: In this classic arcade game, the AI agent had to learn everything from the raw pixel input, without any prior knowledge of the game's objective or controls. After just 100 games, the agent started to grasp the basic concept of moving the bat to hit the ball. By 300 games, it had reached human-level performance. But the most remarkable discovery came after 500 games, when the agent found an optimal strategy that even the researchers had never seen before - digging a tunnel around the side of the wall to put the ball behind it. This demonstrated the AI's ability to explore and discover novel solutions that surpass human expertise.
AlphaGo: The game of Go has long been considered a significant challenge for AI, due to its immense complexity and the importance of strategic long-term thinking. AlphaGo, developed by DeepMind, tackled this challenge by combining a policy network to propose promising moves, a value network to evaluate board positions, and a tree search algorithm to explore future variations. This combination allowed AlphaGo to not only match the best human players but also to discover new, unconventional moves that surprised even the experts. The AI's ability to see the "big picture" and make strategic decisions that had long-term implications was a true breakthrough.
These examples showcase the power of reinforcement learning in enabling AI systems to surpass human performance and discover novel solutions. By learning through trial and error, these agents were able to explore a vast space of possibilities and uncover strategies that were previously unknown, demonstrating the immense potential of this approach for future AI advancements.
Het ontsluiten van het potentieel van grote taalmodellen met Reinforcement Learning
Het ontsluiten van het potentieel van grote taalmodellen met Reinforcement Learning
Reinforcement learning is a powerful machine learning framework that allows AI systems to learn from their own trials and errors. By providing rewards or penalties based on the outcomes of their actions, reinforcement learning agents can develop sophisticated strategies and discover novel solutions to complex problems.
One of the key components of a reinforcement learning system is the policy network, which proposes the actions the agent should take in a given state. This is analogous to a large language model, which can be trained to generate high-quality responses to prompts. By combining the strengths of large language models and reinforcement learning, researchers believe it may be possible to significantly improve the reasoning and logic capabilities of these models.
The hypothesis is that a policy network could be used to propose a set of candidate solutions or steps to solve a problem, while a separate value network could be used to evaluate the quality of these proposals and provide feedback to the policy network. This iterative process of proposal and evaluation could allow the system to explore a wider range of potential solutions and discover novel strategies, much like the AlphaGo system developed by DeepMind.
While the details of the "QAR" breakthrough mentioned in the transcript are still speculative, this general approach of leveraging reinforcement learning to enhance large language models is an exciting area of research. By combining the strengths of these two powerful AI techniques, researchers may be able to unlock new levels of intelligence and problem-solving capabilities in these models.
Conclusie
Conclusie
The potential of reinforcement learning in large language models is an exciting prospect. By incorporating policy networks to propose high-quality solutions and value networks to evaluate the long-term outcomes, the reasoning and logic capabilities of these models could be significantly enhanced.
The ability to explore a wide range of strategies and discover novel solutions, as demonstrated by AlphaGo, suggests that this approach could unlock new problem-solving capabilities in large language models. The "let's verify step-by-step" method, where a separate model critiques the reasoning process, also shows promise in improving the model's internal logic.
While the specifics of OpenAI's "QAR" breakthrough remain uncertain, the general principles of reinforcement learning offer a compelling path forward for advancing the state-of-the-art in large language models. As the field continues to evolve, we can expect to see more exciting developments in this direction, potentially leading to AI systems with unprecedented problem-solving abilities.
FAQ
FAQ