Did xAI Cheat? Investigating the Truth About Grok-3's Benchmarks
Did xAI Cheat? Investigating the Truth About Grok-3's Benchmarks. A detailed analysis of the claims that Grok-3 may have cheated on its benchmarks, and a comparison of its capabilities to the O3 Mini model.
24 febbraio 2025
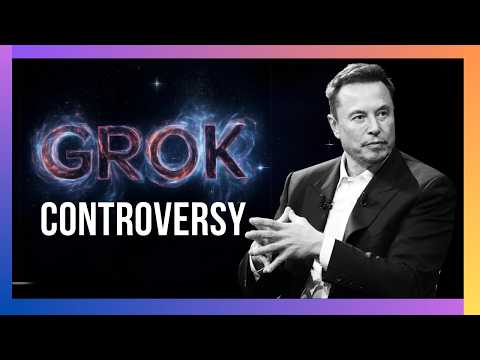
Discover the truth behind the benchmarks of Grok-3, the latest AI model, and learn how it compares to other leading models in terms of reasoning and problem-solving capabilities. This blog post provides an in-depth analysis of Grok-3's performance, highlighting its strengths and potential areas for improvement.
The Controversy Around Grok-3's Benchmarks
Analyzing the Benchmark Results
Grok-3's Reasoning Capabilities
Solving Logical Puzzles with Grok-3
Comparing Grok-3 to Other Models
The Controversy Around Grok-3's Benchmarks
The Controversy Around Grok-3's Benchmarks
The author presents a compelling argument that the benchmarks reported for Grok-3 may have been inflated due to the use of majority voting across multiple runs. They highlight that the Open AI team used a similar technique when reporting results for GPT-3 and O3-mini, which raises concerns about the transparency and fairness of the evaluation process.
The author also notes that when directly comparing the performance of O3-mini on high-settings against a single pass of Grok-3 beta, O3-mini appears to outperform Grok-3. This suggests that the claimed superiority of Grok-3 may not be as substantial as initially reported.
However, the author acknowledges that Grok-3 is still in beta, and the full version may be able to outperform O3-mini without relying on majority voting. They also highlight the impressive reasoning capabilities of Grok-3, particularly in solving modified versions of well-known paradoxes and problems, which sets it apart from other language models.
Overall, the author presents a balanced and nuanced perspective on the ongoing debate surrounding the benchmarks and capabilities of Grok-3, acknowledging both its strengths and the potential issues with the reported results.
Analyzing the Benchmark Results
Analyzing the Benchmark Results
The discussion highlights several key points regarding the benchmark results for Grok 3 and O3 mini:
-
Majority Voting: The original Grok 3 results used a majority vote across 64 different runs, which provided a significant performance boost. This was similar to the approach used by OpenAI when reporting results for GPT-3 and O3 mini.
-
Single-Pass Comparison: When directly comparing O3 mini on high settings with a single pass of Grok 3 beta, O3 mini outperforms Grok 3 on the benchmarks reported by the XAI team.
-
External Validation: The Elo score on the ChatBot Arena leaderboard shows that Grok 3 is the first model to cross the 1400 mark, which is a substantial improvement compared to other models. This external validation suggests that Grok 3's real-world performance is likely much better than the benchmark results.
-
Reasoning Capabilities: The analysis of Grok 3's reasoning capabilities using the Misguided Attention prompts demonstrates its impressive ability to identify and respond to subtle changes in well-known paradoxes and questions. Grok 3 with the "thinking" mode outperforms the standard Grok 3 model in these tasks.
-
Ongoing Development: The discussion notes that Grok 3 is still in beta, and the full version will likely be able to outperform O3 mini without the need for majority voting. The final performance of the two models remains to be seen as development continues.
Overall, the analysis suggests that Grok 3 is a highly capable model, with strong reasoning abilities and external validation of its performance. However, the benchmark results require careful interpretation, and the final comparison between Grok 3 and O3 mini may depend on the continued development of both models.
Grok-3's Reasoning Capabilities
Grok-3's Reasoning Capabilities
Grok-3 has demonstrated impressive reasoning capabilities, particularly when it comes to solving modified versions of well-known paradoxes and problems. The model is able to identify the changes in the problem statements and adapt its reasoning accordingly, often outperforming other language models.
One key aspect of Grok-3's reasoning is its ability to break down the problem, highlight the relevant facts, and then carefully consider the different options and their moral/ethical implications before arriving at a final decision. This is exemplified in its handling of the modified trolley problem, where it recognizes that the five people on the track are already dead and, therefore, pulling the lever would only result in the unnecessary death of the one person on the other track.
Similarly, Grok-3 is able to identify the changes in the Monty Hall problem and the Schrödinger's cat scenario, and provide the correct reasoning and solutions. Its ability to formulate a mathematical representation of the problem, as seen in the modified version of the Russell's Paradox, is also noteworthy.
Overall, Grok-3's reasoning capabilities are a significant strength, and it has demonstrated the ability to tackle complex logical and ethical problems with a level of nuance and depth that sets it apart from many other language models.
Solving Logical Puzzles with Grok-3
Solving Logical Puzzles with Grok-3
Grok-3 is an impressive language model that demonstrates strong reasoning capabilities, particularly in solving logical puzzles and paradoxes. When presented with modified versions of well-known thought experiments, Grok-3 is able to identify the changes and provide thoughtful, nuanced responses.
In the case of the modified trolley problem, where the five people on the main track are already dead, Grok-3 recognizes this key detail and concludes that pulling the lever would only result in the unnecessary death of the one living person on the other track. Its internal thought process is detailed and highlights the relevant facts, leading to a well-reasoned decision.
Similarly, when faced with a variant of the Monty Hall problem, where the host opens the door the contestant had initially chosen, Grok-3 carefully re-reads the prompt and correctly deduces that the probability of winning the car is now 50/50, unlike the classic Monty Hall problem.
The model's ability to identify and adapt to changes in the classic paradoxes, such as Schrodinger's cat being already dead, and the modified version of the barber paradox, is particularly impressive. Grok-3 does not simply refer back to the original problems but formulates new, tailored responses based on the unique details provided.
Overall, Grok-3's performance on these logical puzzles demonstrates its strong reasoning capabilities and its potential to tackle complex, nuanced problems. The model's detailed internal thought processes and its ability to recognize and respond to subtle changes in the prompts set it apart from many other language models.
Comparing Grok-3 to Other Models
Comparing Grok-3 to Other Models
Grok-3 is a highly impressive model, demonstrating strong reasoning capabilities that set it apart from many other language models. When compared to other models, Grok-3 stands out in several key areas:
-
Logical Reasoning: Grok-3's "thinking" mode allows it to effectively identify and address changes or twists in well-known logical puzzles and paradoxes, such as the modified versions of the Trolley Problem, Monty Hall Problem, and Barber Paradox. It is able to break down the scenarios, highlight the key facts, and arrive at the correct conclusions, showcasing its strong logical reasoning skills.
-
Transparency of Thought Process: Grok-3's internal thought process is highly transparent, with the model providing detailed explanations of its reasoning and decision-making. This level of transparency is valuable for understanding the model's capabilities and limitations.
-
Performance on Benchmarks: While there have been some claims of potential benchmark manipulation by the Grok team, the external validation signals, such as the Chatbot Arena leaderboard, suggest that Grok-3 is a highly capable model, outperforming many of its competitors.
-
Adaptability: Grok-3 has demonstrated the ability to adapt to changes and modifications in well-known problems, rather than simply relying on its training data. This adaptability is a valuable trait in real-world applications.
It is important to note that Grok-3 is still in beta, and the full release version may further improve upon its already impressive capabilities. As the model continues to evolve, it will be interesting to see how it compares to other state-of-the-art language models, particularly in areas such as deep search and knowledge retrieval.
FAQ
FAQ