Exploring the Capabilities of OpenAI's GPT-4.1: A Powerful AI Assistant
Explore the impressive capabilities of OpenAI's latest GPT-4.1 model, a powerful AI assistant that excels at coding tasks and benchmarks. Discover how it compares to other cutting-edge AI systems and the challenges of testing their true capabilities.
17 avril 2025

Discover the remarkable advancements in AI technology with this in-depth exploration of OpenAI's latest GPT 4.1 model. Uncover its impressive capabilities, from coding tasks to contextual understanding, and learn how it compares to other cutting-edge AI assistants. This comprehensive overview provides valuable insights for anyone interested in the rapidly evolving world of artificial intelligence.
Discover the Power of GPT 4.1: A Coding-Focused AI Assistant
Navigating the Pareto Frontier: Balancing Speed and Intelligence
Benchmarks: A Double-Edged Sword for AI Evaluation
Humanity's Last Exam: A True Test for AI Capabilities
The Race for AI Supremacy: Competition and Innovation Abound
The Challenges of Training Powerful AI Systems
Unleashing the Potential of Limited Data: Lessons from the Human Brain
Conclusion
Discover the Power of GPT 4.1: A Coding-Focused AI Assistant
Discover the Power of GPT 4.1: A Coding-Focused AI Assistant
GPT 4.1 is a powerful AI assistant that excels in coding tasks, outperforming even the previous GPT 4.5 model. With a context window of 1 million tokens, it can handle vast amounts of information, making it a versatile tool for a wide range of applications.
One of the key advantages of GPT 4.1 is its speed and efficiency. While it may not be as intelligent as the more advanced models, it is optimized for tasks that require rapid response, such as text autocomplete. This makes it an excellent choice for developers who need a fast and reliable coding assistant.
In terms of coding benchmarks, GPT 4.1 has demonstrated impressive performance, often outperforming slower, more sophisticated AI systems. This is a testament to the ongoing advancements in AI technology and the dedication of the teams behind these models.
However, it's important to note that benchmarks have their limitations, as these AI assistants are often trained on vast amounts of data that may include similar content to the test questions. To truly assess the capabilities of these models, more rigorous testing, such as the Humanity's Last Exam, is necessary.
Overall, GPT 4.1 is a valuable addition to the AI landscape, offering a coding-focused assistant that combines speed, efficiency, and impressive performance on a wide range of tasks. As the competition in the AI field continues to intensify, we can expect to see even more impressive advancements in the near future.
Benchmarks: A Double-Edged Sword for AI Evaluation
Benchmarks: A Double-Edged Sword for AI Evaluation
The problem with benchmarks is that they often ask questions that current AI systems already know the answers to, as they are trained on vast amounts of internet data. This means that these benchmarks become less and less meaningful over time, as the AI models can simply regurgitate information they've already seen.
To address this issue, the paper "Humanity's Last Exam" introduces a new approach. The authors asked the smartest people in the world to create questions that none of the current AI systems can answer. These questions span a wide range of disciplines, from classics to ecology, mathematics, computer science, and more. The results were stunning - the AI models, including the newer ones like GPT-4.1, failed spectacularly on this test.
However, the authors of "Humanity's Last Exam" have taken steps to ensure that this test is not easily gamed. Many of the questions are reserved in a hidden dataset that is not published, making it more difficult for the AI models to simply memorize and regurgitate the answers.
This suggests that private datasets, like the one used in "Humanity's Last Exam," might be a more reliable way to measure the true capabilities of AI systems in the future. As the competition between AI labs intensifies, with models like Google DeepMind's Gemini 2.5 Pro emerging as powerful alternatives, it will be important to continue testing these systems on more challenging and unpredictable benchmarks.
Humanity's Last Exam: A True Test for AI Capabilities
Humanity's Last Exam: A True Test for AI Capabilities
The problem with existing AI benchmarks is that they often test on questions that the models have already seen during training. To truly assess the capabilities of these systems, researchers have introduced "Humanity's Last Exam" - a set of questions curated by the world's top experts that are designed to be beyond the current abilities of AI.
These questions span a wide range of disciplines, including classics, ecology, mathematics, computer science, linguistics, and chemistry. The key difference is that these questions are not part of the training data, making it impossible for the models to simply "memorize" the answers.
When tested on Humanity's Last Exam, the latest AI models, including GPT-4.1, have been found to "fail spectacularly." Even the powerful Gemini 2.5 Pro from Google DeepMind struggles with these challenging questions. This suggests that while these AI systems excel at tasks they have been trained on, they still lack the true general intelligence and reasoning abilities to tackle completely novel problems.
Importantly, the researchers have kept a hidden dataset of these questions, ensuring that they cannot be easily incorporated into future training. This makes Humanity's Last Exam a more reliable and truthful measure of AI progress, as the models cannot simply "game" the system by memorizing the questions.
As the field of AI continues to advance rapidly, it will be crucial to have robust and unbiased tests like Humanity's Last Exam to accurately assess the capabilities of these systems. By pushing the boundaries of what AI can do, we can better understand the strengths and limitations of these technologies and guide their development towards true general intelligence.
The Race for AI Supremacy: Competition and Innovation Abound
The Race for AI Supremacy: Competition and Innovation Abound
The landscape of AI is rapidly evolving, with a breakneck pace of innovation and fierce competition between leading labs. The recent release of GPT-4.1, along with the mini and nano models, showcases the remarkable progress in AI capabilities, particularly in the realm of coding and task-specific performance.
These new models form a Pareto frontier, allowing users to choose the right balance between speed and intelligence based on their needs. For tasks like text autocomplete, the nano model may be sufficient, while the regular GPT-4.1 shines in more complex applications like the flash card app mentioned.
Surprisingly, the GPT-4.1 model has even outperformed the previous GPT-4.5 on coding benchmarks, demonstrating the rapid advancements in AI's problem-solving abilities. The context window has also been expanded to an impressive 1 million tokens, enabling AI systems to draw upon a vast knowledge base to tackle complex queries.
However, the speaker cautions against over-reliance on benchmarks, as they may become less meaningful as AI models are trained on increasingly comprehensive datasets. To truly assess the capabilities of these systems, the speaker highlights the importance of tests like "Humanity's Last Exam," which challenge AI with questions that humans find exceptionally difficult.
The competition between AI labs, such as OpenAI, Google DeepMind, and DeepSeek, has resulted in a wealth of powerful and often free-to-use AI models for the public to explore and utilize. This breakneck pace of innovation is fueled by the realization that data has become the bottleneck, rather than compute power, driving the need for more efficient and ingenious approaches to AI training and development.
As the AI landscape continues to evolve, the speaker emphasizes the importance of ongoing testing and evaluation to truly understand the capabilities and limitations of these advanced systems. The future of AI holds immense promise, and the race for supremacy is sure to captivate the scientific community and the public alike.
The Challenges of Training Powerful AI Systems
The Challenges of Training Powerful AI Systems
Training powerful AI systems like GPT-4.1 is an incredibly complex and challenging task. As the models become more advanced, the training process becomes exponentially more demanding.
The key challenge is that the training data required for these models is growing rapidly, but the compute power needed to effectively utilize this data is growing even faster. This has made data the primary bottleneck, rather than compute power.
To address this, AI researchers are looking to the human brain for inspiration. The human brain is remarkably data-efficient, able to learn complex tasks from limited data. The goal is to develop training techniques that can squeeze out every drop of information from the available data, much like how a human can deeply understand a subject from a small textbook.
However, this is easier said than done. The training process is fraught with small bugs and issues that can quickly snowball and derail the entire system. These minor problems, which may be insignificant in simpler systems, become magnified a hundredfold in the highly complex AI models of today.
Overcoming these challenges requires immense ingenuity and a deep understanding of the underlying principles of machine learning. As the competition between AI labs heats up, the pace of innovation is accelerating rapidly. The result is a flood of powerful, often free, AI models that are pushing the boundaries of what was previously thought possible.
Unleashing the Potential of Limited Data: Lessons from the Human Brain
Unleashing the Potential of Limited Data: Lessons from the Human Brain
The key insight here is that the current AI landscape is shifting from being compute-constrained to being data-constrained. While compute power continues to grow at a rapid pace, the availability of high-quality training data has become the new bottleneck. This realization has led to a renewed focus on data efficiency, and the human brain has emerged as a prime example of a highly data-efficient system.
The analogy presented is that of a student facing an exam with a textbook that only contains two problems, while the exam itself has a hundred questions. The solution is not to simply memorize the two problems, but rather to deeply understand the fundamental principles, methods, and reasoning behind them. This approach allows the student to apply the acquired knowledge to solve the remaining 98 problems.
Similarly, the goal for AI systems is to move beyond simply memorizing patterns in the available data and instead develop a deeper understanding of the underlying principles and reasoning. This shift towards data efficiency and knowledge extraction is crucial, as the training of these complex AI models is fraught with small bugs and issues that can quickly escalate into significant problems when scaled up.
The landscape of AI is changing rapidly, with fierce competition between various labs and the continuous release of new, highly capable models. This breakneck pace of innovation is a gift to humanity, providing us with an abundance of powerful AI tools, often for free or at a low cost. However, the true challenge lies in developing the necessary human ingenuity to make the most effective use of the available data and resources, much like the student who understands the principles behind the limited textbook problems.
In summary, the key message is that the future of AI lies in data efficiency and the ability to extract deep insights from limited data, drawing inspiration from the remarkable capabilities of the human brain. As the field continues to evolve, the focus must shift towards leveraging the vast computational resources to uncover the fundamental principles and reasoning that underlie the available data.
Conclusion
Conclusion
The rapid advancements in AI models like GPT-4.1, mini, and nano demonstrate the breakneck pace of innovation in this field. While benchmarks can be useful, they have limitations as the models are often trained on similar data. The "Humanity's Last Exam" approach, with hidden datasets, provides a more robust way to assess the true capabilities of these systems.
The competition between AI labs, such as OpenAI, Google DeepMind, and DeepSeek, has resulted in a wealth of powerful and often free-to-use models. However, the training of these models has become increasingly challenging, with small issues magnified due to the complexity of the systems.
The key focus now is on data efficiency, as compute power is growing faster than the available data. Drawing inspiration from the human brain's ability to learn from limited information, the next chapter for AI will be about leveraging existing data more effectively through improved techniques and ingenuity.
Overall, the AI landscape is evolving rapidly, and the user community is being spoiled with an abundance of capable and often free-to-use models. This is just the beginning of humanity's AI journey, and the future holds even more exciting advancements.
FAQ
FAQ
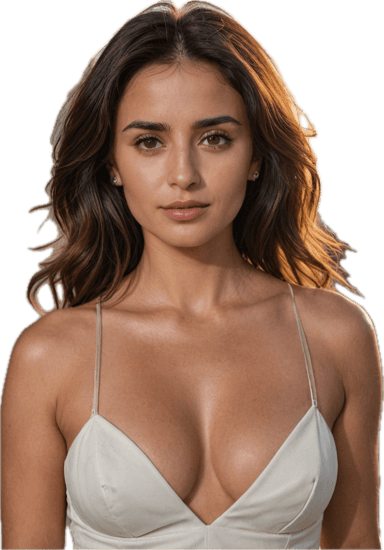
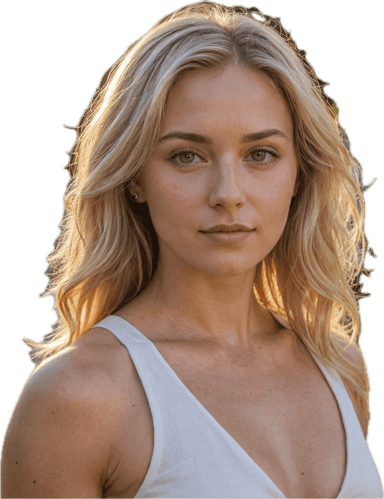