Unleash the Power of AlphaFold 3: Revolutionizing Protein Folding, Enzyme Engineering, and Beyond
Unleash the Power of AlphaFold 3: Revolutionizing Protein Folding, Enzyme Engineering, and Beyond. Discover how this groundbreaking AI model is transforming fields like drug design, bioremediation, and genomics research.
April 22, 2025
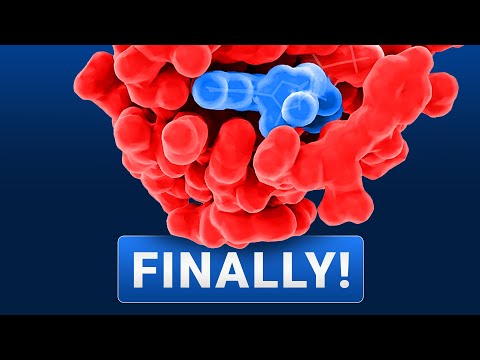
Unlock the power of protein folding with AlphaFold 3, a groundbreaking AI system that revolutionizes drug discovery, renewable material development, and more. Discover how this cutting-edge technology is poised to transform industries and save lives.
The Groundbreaking Potential of AlphaFold 3
Protein Folding: The Building Blocks of Life
Evolving Enzymes to Tackle Plastic Waste
Accuracy Improvements in AlphaFold 3
Predicting Ligand Interactions and Beyond
The Architectural Changes in AlphaFold 3
Limitations and Future Improvements
Explore AlphaFold 3 for Yourself
The Groundbreaking Potential of AlphaFold 3
The Groundbreaking Potential of AlphaFold 3
AlphaFold 3, the latest iteration of the groundbreaking protein folding AI developed by Google DeepMind, represents a significant leap forward in our understanding and manipulation of the building blocks of life. This new model not only outperforms its predecessor in predicting the 3D structures of proteins, but it also extends its capabilities to accurately model the interactions between proteins and small molecules, known as ligands.
The ability to accurately predict the 3D structures of ligands is a game-changer, as it opens up new avenues for drug discovery, the development of biorenewable materials, and advancements in genomics research. AlphaFold 3's performance in this area surpasses even the specialized physics-based systems that have been the industry standard, demonstrating the power of machine learning to tackle complex problems.
Moreover, the model's versatility extends beyond proteins and ligands, as it can now also predict the structures of ions, DNA, and RNA. This multifaceted capability is a testament to the generalist approach of the AlphaFold team, which has enabled the model to outperform specialist systems in their own domains.
The technical advancements behind AlphaFold 3 are equally impressive, with the introduction of the Pairformer module and the diffusion-based approach to generating 3D molecular structures. These innovations, combined with the continued refinement of the model's architecture, have resulted in a significant boost in accuracy, particularly for protein antibodies, where the model's performance has more than doubled.
While the model still has limitations, such as its inability to capture dynamic behaviors and the sensitivity of its diffusion module to the initial random noise, the overall impact of AlphaFold 3 is undeniable. This work is already saving lives and will continue to do so in the future, as it accelerates drug discovery, enables the development of more resilient crops, and contributes to a wide range of other applications that will benefit humanity.
Protein Folding: The Building Blocks of Life
Protein Folding: The Building Blocks of Life
Proteins are the fundamental building blocks of life, composed of a string of amino acids that fold into intricate three-dimensional structures. Determining the precise 3D structure of a protein, known as protein folding, has long been a significant challenge in biology and biochemistry.
The advent of AlphaFold, a groundbreaking AI system developed by Google DeepMind, has revolutionized this field. AlphaFold is capable of accurately predicting the 3D structure of proteins based on their amino acid sequence, a task that was previously considered brutally hard and unsolvable.
The latest iteration, AlphaFold 3, has taken this technology even further. Not only has it improved the accuracy of protein structure prediction, but it can now also handle the prediction of ligands, small molecules that interact with proteins. This capability is crucial for drug discovery and development, as the majority of drugs are small molecules that target specific proteins.
AlphaFold 3's ability to outperform specialized physics-based systems in predicting protein-ligand interactions is a remarkable achievement. This breakthrough has the potential to accelerate the development of new drugs, as well as enable advancements in areas such as biorenewable materials, crop resilience, and genomics research.
The success of AlphaFold 3 is a testament to the power of generalist AI models, which can outperform specialist systems in their own domains. This work is not just about proteins; it represents a significant step towards a future where a single AI system can tackle a wide range of tasks, revolutionizing fields like drug discovery and beyond.
Evolving Enzymes to Tackle Plastic Waste
Evolving Enzymes to Tackle Plastic Waste
This project was enabled by AlphaFold and is about evolving enzymes that can digest plastics. These enzymes are designed to break down these plastics, which helps with recycling them so they can be used over and over again, similar to how glass or tin cans can be recycled infinitely. This eliminates the need to produce more plastics, which requires fossil fuels, and allows for the cleanup of plastic landfills. This breakthrough, published in 2021, sounds like something out of a science fiction movie, but it is a real-world solution that has the potential to significantly impact the global plastic waste problem.
Accuracy Improvements in AlphaFold 3
Accuracy Improvements in AlphaFold 3
AlphaFold 3 has demonstrated significant improvements in accuracy compared to its predecessor, AlphaFold 2. The most notable enhancement is in the prediction of protein antibodies, where the accuracy has more than doubled. This is an impressive achievement, as accurately predicting the 3D structure of protein antibodies is crucial for various medical and biological applications.
Furthermore, AlphaFold 3 has expanded its capabilities beyond just proteins. It can now accurately predict the 3D structures of ligands, small molecules that interact with proteins, as well as ions, DNA, and RNA. This is a remarkable advancement, as the ability to predict the structures of these molecules is essential for drug design, genomics research, and the development of biorenewable materials.
Interestingly, AlphaFold 3 has outperformed the specialized physics-based systems that are widely used in industry for predicting protein-ligand interactions. This is a significant milestone, as it demonstrates the power of AI-based approaches in tackling complex problems that were previously the domain of specialized, physics-based methods.
Predicting Ligand Interactions and Beyond
Predicting Ligand Interactions and Beyond
Ligands are small molecules that interact with proteins, and the majority of drugs are small molecules like these. Previously, AlphaFold was limited to only proteins and could not predict the interactions of proteins with ligands. However, AlphaFold 3 can now not only deal with these ligand molecules, but it also outperforms the specialist physics-based systems that are widely used in industry.
This is a significant breakthrough, as AlphaFold 3 can now predict the 3D structure of ligands, ions, DNA, and RNA with accuracy that surpasses the capabilities of previous methods. This new capability can help accelerate drug design, genomics research, and the development of biorenewable materials and more resilient crops.
The key to this advancement is the new Pairformer module, which has replaced the previous Evoformer module. Additionally, the inclusion of a diffusion module, similar to those used in text-to-image models, enables the creation of 3D molecular structures from a starting point of noise.
This multi-tasking AI system that outperforms specialist models in their own domains is a testament to the power of generalist AI models. The implications of this work are far-reaching, and it is likely that this paper is already saving lives and will continue to do so in the future.
The Architectural Changes in AlphaFold 3
The Architectural Changes in AlphaFold 3
The previous version of AlphaFold, AlphaFold2, utilized an Evoformer module that represented the protein folding problem as a graph, where the nodes represented the amino acid residues and the connections represented their relationships. In AlphaFold 3, this Evoformer module has been replaced with a simpler module called Pairformer.
Additionally, AlphaFold 3 introduces a new diffusion module, which is somewhat similar to the ones used in text-to-image models. This diffusion module starts from a bunch of noise and reorganizes it over time to create the 3D molecular structures, rather than directly predicting the structures.
These architectural changes, along with various other hand-engineered additions, have led to incremental improvements in the accuracy of AlphaFold 3, particularly in the prediction of protein antibodies, where the accuracy has more than doubled compared to the previous version.
Furthermore, AlphaFold 3 has expanded its capabilities beyond just proteins, and can now also accurately predict the 3D structures of ligands, ions, DNA, and RNA. This allows the model to assist in the development of biorenewable materials, more resilient crops, accelerated drug design, and genomics research.
Limitations and Future Improvements
Limitations and Future Improvements
The new AlphaFold 3 model represents a significant advancement in protein structure prediction, but it still has some limitations that the researchers acknowledge.
One key limitation is that the model can only predict static protein structures and cannot capture more dynamic behaviors. This means it may not be able to fully account for the complex conformational changes that proteins can undergo during their biological functions.
Additionally, the diffusion module used in AlphaFold 3 starts from random noise, which can introduce some sensitivity to the initial conditions. This means that different runs of the model may produce slightly different solutions, with varying levels of accuracy. To mitigate this, the researchers recommend running the model multiple times from different starting points and selecting the most accurate prediction.
Looking to the future, the researchers express hope that even simpler and more elegant techniques can be developed to perform these complex protein structure prediction tasks. They note that the current approach, while effective, still involves a significant amount of hand-engineering and the addition of many small improvements. Developing a more streamlined and fundamental approach could lead to further advancements in this field.
Overall, while AlphaFold 3 represents a remarkable achievement, the researchers acknowledge that there is still room for improvement and continued innovation in this rapidly evolving area of computational biology.
Explore AlphaFold 3 for Yourself
Explore AlphaFold 3 for Yourself
The AlphaFold Server provides a free and accessible way for anyone to explore the capabilities of AlphaFold 3. Even if you are not a biologist, the server offers a variety of presets that allow you to easily visualize and interact with the predicted 3D structures of proteins, ligands, ions, DNA, and RNA.
One of the highlights of the AlphaFold Server is its support for dark mode, which provides a visually appealing and comfortable experience for users. The server's intuitive interface makes it easy to navigate and explore the predicted structures, allowing you to gain a deeper understanding of the advancements made in protein folding and molecular structure prediction.
Whether you're a researcher, a student, or simply someone interested in the latest developments in computational biology, the AlphaFold Server is an invaluable resource that allows you to directly engage with the groundbreaking work of AlphaFold 3. Dive in and explore the possibilities for yourself!
FAQ
FAQ