AI Innovations Unveiled: Text-to-Video, Robotics, and Cutting-Edge Models
Discover the latest AI innovations unveiled, including text-to-video models, robotics advancements, and cutting-edge language models. Explore the potential of these breakthroughs and their impact on technology and everyday life.
February 16, 2025
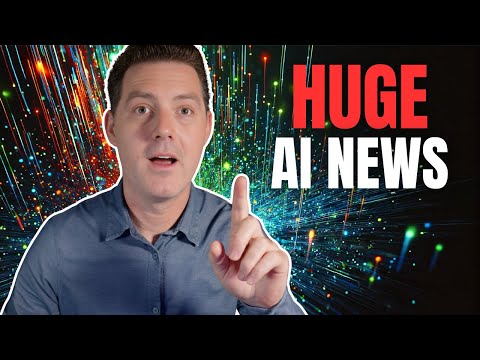
Discover the latest advancements in AI, from cutting-edge text-to-video models to groundbreaking robotics and coding capabilities. This comprehensive overview covers the most significant AI developments, equipping you with the knowledge to stay ahead of the curve.
Unlock the Power of Text-to-Video: Discover the Latest AI Advancements
Runway Gen3: Unleashing a New Era of AI-Powered Video Generation
Unraveling the Truth: Clarifying Apple's AI Announcements and Partnerships
NVIDIA's Nitron 340B: A Groundbreaking Model for Synthetic Data Generation
Cloning Human Motion: Robotic Systems Powered by Real-Time Shadowing
Simulating the Mind of a Rat: Insights from DeepMind and Harvard's Virtual Rodent
Open AI's Cyber Security Expertise: A Move Towards Regulatory Capture?
Stable Diffusion 3: Exploring the Latest Advancements in Text-to-Image AI
Humanoid Drivers: A Novel Approach to Autonomous Vehicles from Japan
Deepseek Coder V2: Dominating the Coding and Math Landscape
Unlock the Power of Text-to-Video: Discover the Latest AI Advancements
Unlock the Power of Text-to-Video: Discover the Latest AI Advancements
The world of AI has been abuzz with exciting developments, and the advancements in text-to-video technology are particularly noteworthy. Two new models, Luma AI's "Dream Machine" and Runway's "Gen 3 Alpha," have emerged as impressive contenders in this rapidly evolving field.
Luma AI's "Dream Machine" allows users to generate stunning videos from text or image prompts. The level of detail and physics-based interactions in the resulting videos is truly remarkable, with characters, objects, and environments seamlessly blending together. While the model still struggles with certain aspects like text rendering and morphing, the overall quality is a significant step forward in the text-to-video landscape.
Runway's "Gen 3 Alpha" is another impressive addition to the text-to-video arena. The model showcases a wide range of capabilities, from creating realistic-looking people and creatures to generating detailed scenes with intricate lighting, reflections, and camera movements. The side-by-side comparison with Sora's previous work highlights the impressive advancements made by Runway's latest offering.
These new models not only push the boundaries of what's possible in text-to-video generation but also raise the bar for open-source alternatives. The lack of readily available open-source text-to-video models that can compete with the capabilities of these closed-source offerings presents an exciting opportunity for further innovation and collaboration in the AI community.
As the field of text-to-video continues to evolve, the impact of these advancements on various industries, from entertainment to education, is poised to be transformative. The ability to seamlessly translate ideas into visually compelling content holds immense potential, and the continued progress in this domain is sure to captivate and inspire.
Runway Gen3: Unleashing a New Era of AI-Powered Video Generation
Runway Gen3: Unleashing a New Era of AI-Powered Video Generation
Runway, the pioneering company in the text-to-video revolution, has just announced the third version of their groundbreaking AI video generation model, Gen3 Alpha. This latest iteration showcases remarkable advancements, delivering a level of realism and consistency that pushes the boundaries of what's possible in AI-generated video.
The examples provided demonstrate Runway Gen3's exceptional capabilities. From the seamless integration of a wig onto a bald man's head to the lifelike movements of a dragon-toucan hybrid, the model exhibits an uncanny ability to blend the real and the fantastical. The attention to detail is staggering, with the physics of the train's power cables and the reflections in the car window showcasing a deep understanding of the physical world.
One particularly impressive aspect is the direct comparison to Sora, a leading text-to-video model. Runway Gen3 holds its own, delivering results that are on par, if not surpassing, the previous industry standard. This level of competition is a testament to the rapid progress in this field.
Notably, the open-source landscape for text-to-video models remains sparse, with Runway Gen3 and its closed-source counterparts leading the charge. The hope is that an open-source model will soon emerge, providing wider accessibility and further driving innovation in this exciting domain.
Overall, Runway Gen3 represents a significant milestone in the evolution of AI-powered video generation. The level of realism, consistency, and attention to detail showcased in the examples is truly remarkable, setting a new benchmark for the industry. As the technology continues to advance, the possibilities for AI-generated content are poised to expand exponentially.
Unraveling the Truth: Clarifying Apple's AI Announcements and Partnerships
Unraveling the Truth: Clarifying Apple's AI Announcements and Partnerships
Apple's recent AI announcements have generated a lot of confusion and misinformation. Let's set the record straight:
-
Apple has developed its own 3 billion parameter AI model that runs locally on its devices. This model powers various tasks like Siri and other on-device AI capabilities.
-
For more complex queries that require broader knowledge, Apple will prompt the user to send the request to ChatGPT, which is owned and operated by OpenAI. However, this is just an API call, not a deep integration.
-
Contrary to popular belief, OpenAI is not powering or deeply integrated into Apple's core OS and AI functionalities. Apple has its own proprietary cloud-based AI model for these tasks.
-
The partnership with OpenAI is limited to handling certain "world knowledge" queries that Apple's local model cannot address. This is a small subset of the overall AI capabilities Apple has announced.
-
Apple's approach of leveraging its own powerful on-device AI model, while selectively using OpenAI's capabilities, is a strategic move to maintain control and privacy over user data and interactions.
In summary, Apple's AI announcements showcase its commitment to developing robust, privacy-focused AI solutions that can handle a wide range of tasks locally, while selectively tapping into external AI resources when necessary. This balanced approach has been misunderstood by many, leading to unfounded concerns and misinformation.
NVIDIA's Nitron 340B: A Groundbreaking Model for Synthetic Data Generation
NVIDIA's Nitron 340B: A Groundbreaking Model for Synthetic Data Generation
NVIDIA has recently released a massive 340 billion parameter model called Nitron 4 340B. This model is part of a family of models optimized for NVIDIA's Nemo and Tensor RT platforms. Nitron 4 340B includes cutting-edge instruct and reward models, as well as a dataset for generative AI training.
The primary purpose of this model is to serve as a foundation for training smaller models. By generating synthetic data, Nitron 4 340B can help companies and researchers who may not have access to large proprietary datasets to compete more effectively. This is a significant breakthrough, as companies like OpenAI have been paying substantial sums to acquire data from various sources, including Reddit.
With Nitron 4 340B, developers can now generate their own synthetic data to train smaller models, potentially leveling the playing field and allowing more organizations to participate in the AI race. The open-source nature of this model also makes it accessible to a wider audience, further democratizing the development of advanced AI systems.
While the author has not yet had the opportunity to test the model, they are excited to explore its capabilities and potential applications in the near future. The ability to generate high-quality synthetic data could have far-reaching implications for the development of AI models across various industries.
Cloning Human Motion: Robotic Systems Powered by Real-Time Shadowing
Cloning Human Motion: Robotic Systems Powered by Real-Time Shadowing
Research from Stanford has introduced a novel approach called "human-plus" that enables robots to shadow and clone human motion in real-time. This system utilizes a single RGB camera to capture human movements, which are then translated into the corresponding robotic actions.
The key highlights of this system include:
- Real-time cloning of human motion, including complex tasks like boxing, playing the piano, ping-pong, and more.
- Leverages a whole-body policy to accurately replicate the human's movements and interactions with the environment.
- Uses open-source hardware components, including Inspire Robotics hands, Unry Robotics H1 robot body, Dynamixel motors, and Razor webcams.
- Completely open-source design, allowing for easy replication and further development.
This innovative approach demonstrates the potential for robots to seamlessly integrate with and mimic human behavior, paving the way for more natural and intuitive human-robot interactions. By harnessing the power of real-time shadowing, these robotic systems can expand their capabilities and adapt to a wide range of tasks and environments.
Human-plus represents a significant step forward in the field of robotics, showcasing the remarkable progress in bridging the gap between human and machine capabilities.
Simulating the Mind of a Rat: Insights from DeepMind and Harvard's Virtual Rodent
Simulating the Mind of a Rat: Insights from DeepMind and Harvard's Virtual Rodent
DeepMind and Harvard researchers have created a virtual rodent powered by an AI neural network, allowing them to compare real and simulated neural activity. This groundbreaking work represents a significant step towards understanding the complex workings of the mammalian brain.
The researchers used deep reinforcement learning to train the AI model to operate a biomechanically accurate rat model. By doing so, they were able to gain insights into the neural processes underlying the rat's behavior, such as its movements and decision-making.
This virtual rodent simulation not only provides a valuable tool for neuroscientific research but also raises intriguing questions about the potential for scaling up such simulations. If researchers can successfully model the neural activity of a rat, what might be possible when it comes to simulating more complex mammalian brains, including the human brain?
The implications of this research extend beyond the realm of neuroscience. As we continue to push the boundaries of artificial intelligence, the ability to create virtual models that accurately mimic biological systems could have far-reaching applications in fields such as robotics, medicine, and even the development of more advanced AI systems.
Overall, this work from DeepMind and Harvard represents an exciting advancement in our understanding of the mammalian brain and the potential for using AI-powered simulations to unlock its secrets.
Open AI's Cyber Security Expertise: A Move Towards Regulatory Capture?
Open AI's Cyber Security Expertise: A Move Towards Regulatory Capture?
Open AI's announcement of retired US Army General Paul M. Nakasone joining their board of directors is being framed as a move to bring in world-class cyber security expertise. However, this decision raises concerns about potential regulatory capture.
While Open AI is positioning Nakasone's appointment as a way to bolster their cyber security capabilities, it can also be seen as a strategic move to deepen their ties with the security establishment, including the NSA and the military. This could be interpreted as an attempt to gain influence and potentially shape the regulatory landscape surrounding AI development and deployment.
The report that Open AI has a 40-person team dedicated to lobbying Washington further reinforces the notion of regulatory capture. This suggests that the company is actively working to navigate the political and regulatory environment, potentially prioritizing their own interests over broader societal concerns.
Additionally, the rumor that Sam Altman is considering converting Open AI into a for-profit entity raises questions about the organization's true motivations. This shift away from a non-profit structure could further erode public trust, as it may be perceived as a move towards prioritizing financial gain over ethical AI development.
While Open AI's models may continue to be among the best in the industry, the company's actions and decisions are increasingly being viewed with skepticism by the broader AI community. If Open AI continues down this path, they risk losing the trust and goodwill of those who have previously championed their work.
Stable Diffusion 3: Exploring the Latest Advancements in Text-to-Image AI
Stable Diffusion 3: Exploring the Latest Advancements in Text-to-Image AI
Stable Diffusion 3, the latest iteration of the popular text-to-image AI model, has been released by Stability AI. While I have tested it out, I haven't found it to be particularly mind-blowing compared to previous versions. The model seems to perform adequately, but doesn't represent a significant leap forward in capabilities.
That said, if you're interested in exploring Stable Diffusion 3, I'd be happy to create a tutorial on how to set it up on your machine. However, there are already many resources available online that cover the setup process, so I may hold off on creating a tutorial unless there is a strong demand for it from the community.
Overall, Stable Diffusion 3 is a solid text-to-image model, but doesn't seem to offer groundbreaking new features or capabilities. If you're curious to try it out, feel free to let me know, and I'll consider creating a tutorial. Otherwise, you may want to explore other available resources to get started with this latest version of the Stable Diffusion model.
Humanoid Drivers: A Novel Approach to Autonomous Vehicles from Japan
Humanoid Drivers: A Novel Approach to Autonomous Vehicles from Japan
Japan has introduced a novel approach to autonomous vehicles, utilizing humanoid robots as the drivers. In this system, the vehicle itself is a standard automobile, but the driving is performed by a humanoid robot situated within the car.
The humanoid robot is responsible for interpreting the surrounding environment, making driving decisions, and controlling the vehicle's movements. This approach allows for a more natural and intuitive driving experience, as the humanoid robot can mimic human behaviors and reactions behind the wheel.
The research team has published a detailed paper outlining the technical aspects of this system. They have developed a comprehensive framework that enables the humanoid robot to effectively navigate the road, adhere to traffic rules, and safely operate the vehicle.
One of the key advantages of this approach is the ability to leverage the advanced sensory capabilities and decision-making skills of the humanoid robot. By integrating cutting-edge computer vision, object recognition, and motion planning algorithms, the robot can navigate the complex driving environment with precision and adaptability.
Furthermore, the use of a humanoid form factor allows for seamless integration with the vehicle's controls and interfaces, enabling the robot to interact with the car's systems in a natural and intuitive manner.
This innovative approach to autonomous vehicles holds the potential to redefine the future of transportation, blending the capabilities of advanced robotics with the familiarity of traditional automobile design. As the research and development in this field continue, we may witness a paradigm shift in the way we perceive and interact with self-driving vehicles.
Deepseek Coder V2: Dominating the Coding and Math Landscape
Deepseek Coder V2: Dominating the Coding and Math Landscape
Deepseek Coder V2 is the latest iteration of one of the best coding models available. This open-source model has demonstrated impressive performance, outperforming the likes of GPT-4 Turbo, Gemini 1.5 Pro, Claude 3, Opus Llama 370B, and Codstrol across a range of benchmarks.
The key highlights of Deepseek Coder V2 include:
- Beats GPT-4 Turbo, the top coding model, in human evaluation, MBPP+ math, GSAK, and more.
- Excels in both coding and math tasks, showcasing its versatility.
- Supports an impressive 338 programming languages.
- Available in two sizes: a 230 billion parameter version and a smaller 16 billion parameter version.
- Provides API access, allowing for easy integration into various applications.
- Fully open-source, enabling developers to explore and build upon the model.
The performance of Deepseek Coder V2 is truly remarkable, solidifying its position as a leading model in the coding and math domains. With its extensive language support, strong coding and math capabilities, and open-source availability, this model is poised to have a significant impact on the AI-powered coding landscape.
FAQ
FAQ