The Future of AI: From Humanoid Robots to Superintelligence
Explore the future of AI through humanoid robots, superintelligence, and cutting-edge technologies. Learn about advancements in robotics, artificial general intelligence (AGI), and the potential impact on society. Dive into the latest innovations and expert insights shaping the AI revolution.
April 19, 2025
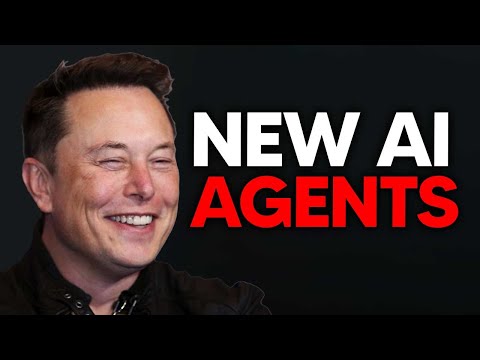
Discover the latest advancements in AI and robotics, from humanoid robots that can mimic human movements to Elon Musk's ambitious plans for Tesla's Optimus robot. Explore the potential of AI agents and how they are pushing the boundaries of what's possible. Stay informed on the evolving landscape of AI and its impact on our future.
Autonomous Humanoid Shadowing: Imitation Learning from Humans
Elon Musk's Vision for Humanoid Robots: 100 Million Units Produced Annually
New AI Agent Jace: Claims and Limitations
Open AI's Appointment of Former NSA Official: Implications for AI Governance
Mixture of Agents Approach Surpasses GPT-4 on Benchmark
Autonomous Humanoid Shadowing: Imitation Learning from Humans
Autonomous Humanoid Shadowing: Imitation Learning from Humans
Stanford University, in collaboration with Google DeepMind, has developed a fascinating new approach to training autonomous robots. The key idea is to enable humanoid robots to observe and mimic human movements in real-time, using an RGB camera to capture the human body and hand motions.
The process involves collecting human motion data using advanced pose estimation algorithms, and then training a policy in a simulation environment to allow the robot to shadow these movements. This "human plus" approach creates a new pipeline for training autonomous robots, leveraging the rich data of human actions.
While the base robot model, the Unitree H1, has limited degrees of freedom compared to the human body, the researchers have been able to train the robot to perform a variety of autonomous tasks, including folding clothes, high jumping, and navigating through a warehouse environment. The robot is able to execute these tasks fully autonomously, without any teleoperation.
The researchers note that the rigid nature of the current hardware platform poses challenges, but they are excited about the potential of applying these techniques to more advanced robotic platforms in the future. The ability to seamlessly integrate human motion data into the training of autonomous robots represents a significant step forward in the field of humanoid robotics and imitation learning.
As the hardware capabilities continue to improve, the researchers hope to explore deploying these autonomous skills on newer, more flexible robotic platforms. This could unlock even more impressive feats of dexterity and versatility, bringing us closer to a future where humanoid robots can truly assist and collaborate with humans in a wide range of tasks.
Elon Musk's Vision for Humanoid Robots: 100 Million Units Produced Annually
Elon Musk's Vision for Humanoid Robots: 100 Million Units Produced Annually
Elon Musk has shared his ambitious vision for the future of humanoid robots. He believes that Tesla will be able to produce 100 million humanoid robots, called the Optimus, per year. Musk envisions these robots being capable of performing a wide range of tasks, from household chores to industrial work.
Musk argues that the demand for these humanoid robots will be immense, with potentially one robot for every human on the planet. He believes the cost of producing these robots at scale could be as low as $10,000 per unit, allowing them to be affordable for both individual consumers and businesses.
While Musk's timeline and production targets may seem overly optimistic, he believes that advancements in artificial intelligence and robotics will make this vision a reality within the next 10-20 years. Musk sees these humanoid robots as a transformative technology that could fundamentally change the way we live and work.
However, Musk's ambitious claims have been met with some skepticism, as he has a history of setting aggressive timelines that are not always met. Nonetheless, the potential of humanoid robots to automate a wide range of tasks is undeniable, and Musk's vision highlights the rapid pace of progress in this field.
As the development of humanoid robots continues, it will be important to closely monitor the ethical and societal implications of this technology, ensuring that it is deployed in a responsible and beneficial manner.
New AI Agent Jace: Claims and Limitations
New AI Agent Jace: Claims and Limitations
Former Meta researchers have released a new AI agent called Jace, which they claim can handle a wide range of tasks autonomously. The demo showcases Jace planning a trip, creating a company, and performing other web-based activities.
While the claims made about Jace's capabilities are impressive, it's important to note a few key limitations:
-
Planning and Multi-Step Reasoning: Current AI agents still struggle with complex planning and multi-step reasoning. The ability to create an LLC from scratch, as claimed in the demo, would require advanced planning and decision-making skills that are not yet widely available in AI systems.
-
Speed and Reliability: The demo acknowledges that Jace's current browsing speed is "somewhat slow" and that the team is working to make it faster and more reliable.
-
Limitations on Complicated Tasks: The creators state that Jace "can struggle with complicated tasks", suggesting there are still significant limitations to its capabilities.
-
Lack of Public Access: Jace is currently in a closed beta, and users can only join the waitlist to access the agent. This limited availability makes it difficult to independently verify the claims made about its abilities.
While the development of Jace is an interesting advancement in the field of AI agents, it's important to approach such claims with a critical eye. The AI community has seen many ambitious promises in the past, and it's crucial to wait for independent verification and further advancements before drawing conclusions about the true capabilities of this or any other AI system.
Open AI's Appointment of Former NSA Official: Implications for AI Governance
Open AI's Appointment of Former NSA Official: Implications for AI Governance
Open AI's recent appointment of a former National Security Agency (NSA) official to its board has raised concerns about the potential implications for AI governance. This move suggests that Open AI is taking steps to address the national security implications of its advanced AI technologies.
The former NSA official, who was previously in charge of mass surveillance programs, is now tasked with providing oversight and guidance to Open AI. This appointment indicates that the company is anticipating increased government scrutiny and regulation of its AI systems, particularly as they become more powerful and capable.
The appointment also raises questions about the balance between innovation and security in the AI field. On one hand, Open AI's advanced AI technologies have the potential to drive significant technological progress and societal benefits. However, there are also valid concerns about the potential misuse of these technologies for surveillance, manipulation, or other nefarious purposes.
By bringing in a former NSA official, Open AI is signaling that it is taking these concerns seriously and is willing to work with government agencies to ensure that its AI systems are developed and deployed responsibly. This move could also be seen as a preemptive measure to avoid potential conflicts with government regulators in the future.
Overall, the appointment of the former NSA official to Open AI's board is a significant development that highlights the growing importance of AI governance and the need for collaboration between the private sector and government agencies to address the complex challenges posed by advanced AI technologies.
Mixture of Agents Approach Surpasses GPT-4 on Benchmark
Mixture of Agents Approach Surpasses GPT-4 on Benchmark
Researchers have introduced a "mixture of agents" approach that leverages multiple large language models (LLMs) to achieve state-of-the-art performance, surpassing even the powerful GPT-4 model.
The key aspects of this approach are:
-
Collective Strength of Multiple LLMs: By harnessing the collective strength of several open-source LLM agents, the researchers were able to improve the overall quality of the responses.
-
Reference Implementation: The researchers provided a reference implementation called "Mixture of the Arts" that utilizes various open-source LLM agents to achieve a score of 65.1% on the Alpaca EV Eval 2.0 benchmark, surpassing the previous leader, GPT-4.
-
Multi-Layer Architecture: The approach organizes the LLM agents into multiple layers, where the outputs from one layer are fed into the next layer for further refinement. This iterative process allows the system to synthesize high-quality responses.
-
Synthesizer/Aggregator: A key component is the "synthesizer" or "aggregator" that combines the responses from the different LLM agents in each layer to produce a single, high-quality output.
The results demonstrate the power of leveraging the collective capabilities of multiple LLMs, even when using open-source models that are not as advanced as GPT-4. This approach highlights the potential for further advancements in large language model performance through innovative architectural designs and ensemble techniques.
FAQ
FAQ