Tiny DeepSeek R1 Clone Outperforms OpenAI GPT-3 in Math - PhD Student's Breakthrough
Breakthrough in AI Math Capabilities: 1.5B Parameter Model Outperforms OpenAI GPT-3. PhD Student's Tiny DeepSeek R1 Clone Demonstrates Efficient Reinforcement Learning for Narrow, High-Performance AI.
23 tháng 3, 2025
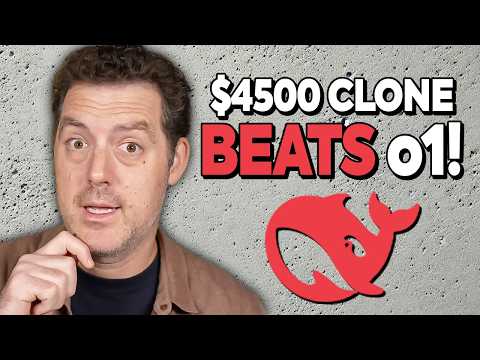
Discover the power of tiny AI models that can outperform their larger counterparts. This blog post explores a groundbreaking discovery where a 1.5 billion-parameter model, trained using distributed reinforcement learning, surpasses the performance of OpenAI's much larger model on a broad range of math tasks. Prepare to be amazed by the efficiency and capabilities of these cutting-edge AI systems.
The Emergence of Tiny, Powerful AI Models
Deep Scaler: Outperforming OpenAI's Massive Model
The Power of Reinforcement Learning and Verifiable Rewards
Distributed Training and Efficiency Gains
Comparing Model Sizes and Accuracy
Outcome vs. Process Reward Models: Implications for Learning
Accessible and Affordable AI Innovations
Hands-on Demonstration: Running Deep Scaler on a Local Machine
Conclusion
The Emergence of Tiny, Powerful AI Models
The Emergence of Tiny, Powerful AI Models
The recent advancements in AI have ushered in the era of tiny, yet powerful models. A team from Berkeley has released a 1.5 billion parameter model, dubbed "Deep Scaler," that outperforms OpenAI's GPT-3.1 in general math tasks. This model, trained using distributed reinforcement learning with verifiable rewards, demonstrates that small-scale models can achieve remarkable performance, challenging the notion that scaling benefits only large models.
The key to this success lies in the use of high-quality data distilled from larger models, which enables smaller models to learn to reason more effectively through reinforcement learning. The Deep Scaler model was trained with just 3,800 A100 GPU hours, a significant reduction compared to the original Deep Seek R1 model, while still surpassing the performance of the much larger OpenAI GPT-3.1 model.
This breakthrough highlights the potential of tiny, narrowly-trained models to excel in specific domains, such as general math tasks. The ability to achieve state-of-the-art results with a 1.5 billion parameter model, which can be easily deployed on mobile devices, opens up new possibilities for efficient and accessible AI applications.
The open-sourcing of the Deep Scaler model and its training pipeline further democratizes this technology, allowing researchers and developers to build upon these advancements and explore the full potential of tiny, powerful AI models.
Deep Scaler: Outperforming OpenAI's Massive Model
Deep Scaler: Outperforming OpenAI's Massive Model
Deep Scaler is a 1.5 billion parameter language model that has been fine-tuned using distributed reinforcement learning. This model has achieved a remarkable feat by outperforming OpenAI's massive 01 Preview model on the AIM 2024 math benchmark, scoring 43.1 - the best in class.
The key advantages of Deep Scaler are its small model size and efficient training process. While 01 Preview is a large and resource-intensive model, Deep Scaler was trained using only 3,800 A100 GPU hours, which is a significant 18.42x reduction compared to the original Deep Seek R1 model. This demonstrates that reinforcement learning can be effectively applied to smaller models, challenging the common myth that RL scaling only benefits large models.
The use of an outcome reward model, as opposed to a process reward model, is a notable aspect of Deep Scaler's training. This approach rewards the model for getting the entire problem correct, rather than providing step-by-step feedback. While the process reward model is generally considered more effective, the outcome reward model used here has still led to impressive results.
The availability of Deep Scaler as an open-source model, with the ability to download the weights and recreate the training pipeline, is a valuable resource for the research community. This allows others to build upon the techniques demonstrated and potentially achieve even greater performance with further refinements.
Overall, Deep Scaler's ability to outperform a much larger model like 01 Preview, while being significantly more efficient to train, is a remarkable achievement that showcases the potential of reinforcement learning and the era of tiny, highly capable models.
The Power of Reinforcement Learning and Verifiable Rewards
The Power of Reinforcement Learning and Verifiable Rewards
Reinforcement learning with verifiable rewards has emerged as a powerful approach to training highly capable models, even with relatively small model sizes. The recent release of the 1.5 billion parameter "Deep Scaler" model from a team at Berkeley is a prime example of this.
The Deep Scaler model, fine-tuned from the Deep Seek R1 distilled Quin 1.5b using distributed reinforcement learning, has achieved state-of-the-art performance on the AIM 2024 math benchmark, outperforming the much larger OpenAI 01 Preview model. This is a remarkable feat, demonstrating that reinforcement learning can enable smaller models to reason more effectively and achieve superior performance on specific tasks.
A key advantage of the reinforcement learning approach used here is the use of an "outcome reward model" rather than a "process reward model". This means the model is rewarded for getting the entire problem right, rather than being rewarded for each individual step. This encourages the model to learn to think through the problem holistically, rather than just memorizing step-by-step solutions.
The authors also highlight that the scaling benefits of reinforcement learning are not limited to large models. Even smaller models, when trained with high-quality data distilled from larger models, can learn to reason more effectively using reinforcement learning. This is exemplified by the $30 Deep Seek clone experiment, as well as the impressive performance of the 1.5 billion parameter Deep Scaler model.
In summary, this work showcases the power of reinforcement learning with verifiable rewards, which can enable the development of highly capable models at a fraction of the cost and size of traditional approaches. The implications of this breakthrough are significant, as it paves the way for the widespread deployment of efficient, task-specific AI models across a wide range of applications.
Distributed Training and Efficiency Gains
Distributed Training and Efficiency Gains
The Deep Scaler model, a 1.5 billion parameter language model, was fine-tuned using a distributed reinforcement learning approach. This distributed approach allowed the model to be trained on longer context lengths, a key factor in its superior performance compared to the larger OpenAI GPT-1 model.
The training of Deep Scaler was highly efficient, requiring only 3,800 A100 GPU hours, a significant 2.42 times reduction compared to the original Deep Seek R1 model. This efficiency was achieved through the use of high-quality SFT (Supervised Fine-Tuning) data distilled from larger models, which enabled the smaller Deep Scaler model to learn to reason more effectively using reinforcement learning.
The total cost of training Deep Scaler was just $4,500, demonstrating the cost-effectiveness of this approach. Furthermore, the model and its training pipeline have been open-sourced, allowing anyone to download the weights and recreate the model themselves.
The quantized version of the Deep Scaler model is only 1.12 GB in size, making it easily deployable and runnable even on modest hardware like the M2 Mac used in the example. This highlights the potential for these tiny, highly capable models to be widely accessible and utilized, ushering in a new era of efficient and powerful AI applications.
Comparing Model Sizes and Accuracy
Comparing Model Sizes and Accuracy
The key points from the transcript are:
- Deep Scaler is a 1.5 billion parameter language model that outperforms the much larger OpenAI GPT-3 model on the AIM 2024 math benchmark, scoring 43.1 compared to GPT-3's 40.0.
- This demonstrates that smaller models trained using distributed reinforcement learning can achieve superior performance on narrow tasks compared to larger general-purpose models.
- The training cost for Deep Scaler was only $4,500, a significant reduction compared to the original Deep Seek R1 model.
- The quantized version of Deep Scaler is only 1.12 GB in size, making it easily deployable on consumer hardware like smartphones.
- The model is able to perform extensive reasoning, using 21,000 tokens to solve a single math problem, showcasing its capabilities despite its small size.
- This highlights the potential for highly specialized, efficiently trained models to outperform larger generalist models on specific tasks.
Outcome vs. Process Reward Models: Implications for Learning
Outcome vs. Process Reward Models: Implications for Learning
The key distinction between outcome reward models and process reward models lies in how the model is incentivized during the learning process. Outcome reward models focus on the final result, providing a reward or penalty based solely on whether the overall task was completed successfully or not. In contrast, process reward models evaluate the model's performance at each step of the task, rewarding it for making the right decisions along the way, even if the final outcome is incorrect.
The advantage of process reward models is that they encourage the model to learn the underlying reasoning and problem-solving skills, rather than simply optimizing for the final answer. By receiving feedback at each step, the model can better understand the thought process required to arrive at the correct solution. This leads to more robust and generalizable learning, as the model develops a deeper understanding of the task rather than relying on surface-level patterns.
In the context of the deep scaler model discussed, the use of an outcome reward model means that the model is only rewarded for getting the entire math problem correct. If it makes mistakes at intermediate steps but arrives at the right final answer, it is still considered successful. This can limit the model's ability to learn the nuanced reasoning required for complex mathematical reasoning.
Conversely, a process reward model would provide feedback at each step of the problem-solving process, allowing the deep scaler model to learn from its mistakes and develop a more comprehensive understanding of mathematical concepts. This approach may lead to slower initial progress, but can result in a more versatile and adaptable model in the long run.
Accessible and Affordable AI Innovations
Accessible and Affordable AI Innovations
The recent advancements in AI have ushered in an era of highly capable yet compact models. The Deep Scaler model, a 1.5 billion parameter language model, has demonstrated its superiority over the much larger OpenAI's GPT-1 model in general math tasks.
This achievement is particularly noteworthy as it was accomplished using a relatively small model that can easily fit on a smartphone and run efficiently. The key to this success lies in the use of distributed reinforcement learning, which allowed the model to be trained on a large scale while keeping the parameter count low.
Furthermore, the researchers have open-sourced the model and its training pipeline, making it accessible for anyone to download and experiment with. This democratization of AI technology is a significant step forward, as it enables more individuals and organizations to leverage powerful AI capabilities without the need for massive computational resources.
The ability to train highly capable models on modest hardware is a testament to the rapid progress in AI research. It paves the way for a future where advanced AI solutions become more widely available and affordable, empowering a broader range of applications and use cases.
Hands-on Demonstration: Running Deep Scaler on a Local Machine
Hands-on Demonstration: Running Deep Scaler on a Local Machine
The Deep Scaler model, a 1.5 billion parameter language model fine-tuned using distributed reinforcement learning, has demonstrated impressive performance on the AIM 2024 math benchmark, surpassing the larger OpenAI 01 preview model. In this section, we will explore the practicality of running this model on a local machine.
The Deep Scaler model is available in two versions: the full F32 precision model, which is 7 GB in size, and the quantized Q5 version, which is only 1.12 GB. For this demonstration, we will be using the Q5 version, which is easily offloadable to a GPU and can be run efficiently on a local machine.
To test the model's capabilities, we will use one of the problems from the AIM 2024 math benchmark. The model processes the problem, generating a significant amount of output as it thinks through the solution, demonstrating its reasoning process. Despite the model's 43% accuracy rating on the benchmark, the level of detail and thought process exhibited in the output is impressive, especially considering the model's small size and the fact that it is running on a local machine while also performing other tasks in the background.
This hands-on demonstration showcases the power and efficiency of the Deep Scaler model, highlighting the potential of small, narrowly-trained models to outperform larger, more general-purpose models in specific domains. The ability to run such a capable model locally, even on a modest machine, opens up exciting possibilities for practical applications and further research in the field of reinforcement learning and efficient model scaling.
Conclusion
Conclusion
The emergence of tiny yet powerful AI models, such as the 1.5 billion parameter "Deep Scaler" model, is a testament to the advancements in reinforcement learning and distributed training techniques. This model, which was fine-tuned from the Deep Seek R1 distilled Quin 1.5b using distributed reinforcement learning, has demonstrated its superiority over the much larger OpenAI GPT-1 model in the realm of general math tasks.
The key highlights of this achievement are:
- The Deep Scaler model outperformed the GPT-1 model on the AIM 2024 math benchmark, achieving a score of 43.1, which is the best in class.
- The model was trained using only 3,800 A100 GPU hours, which is a significant reduction compared to the original Deep Seek R1 training.
- The total cost of training the model was just $4,500, making it an incredibly cost-effective solution.
- The model has been open-sourced, allowing anyone to download the weights and recreate the training pipeline.
- Even the quantized version of the model, which is only 1.12 GB in size, can run efficiently on a local machine, including an M2 Mac, while performing complex mathematical reasoning.
This breakthrough demonstrates the power of reinforcement learning, even with smaller models, and challenges the common myth that scaling benefits only large models. The ability to distill high-quality data from larger models and leverage reinforcement learning to train smaller models effectively has opened up new possibilities in the field of AI.
As the era of tiny yet capable models continues to unfold, we can expect to see more innovative applications and solutions that push the boundaries of what is possible with limited computational resources.
Câu hỏi thường gặp
Câu hỏi thường gặp
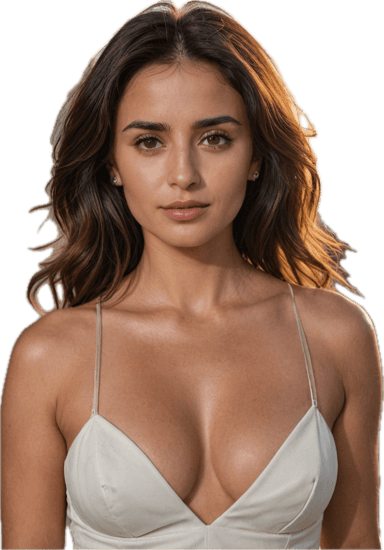
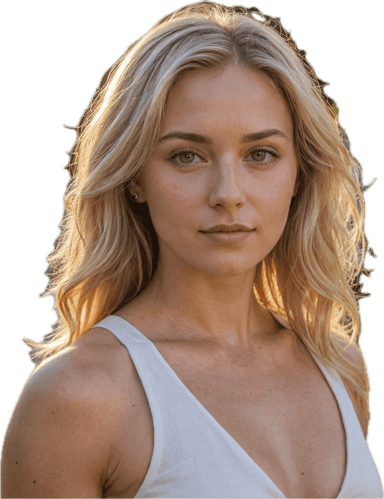