Mastering Reinforcement Learning: Unlocking the Power of Verifiable Rewards
Unleash the Power of Verifiable Rewards in Reinforcement Learning. Discover how aligning AI agents with clear objectives through verifiable rewards can drive predictable, trustworthy behavior and enable models to tackle complex tasks. Explore real-world applications in STEM fields and large language models.
21 aprile 2025
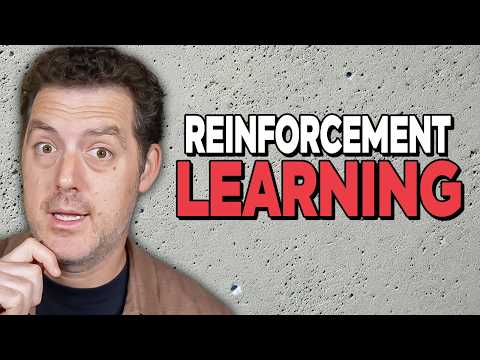
Discover the power of reinforcement learning and how it enables AI models to think and solve complex problems. This blog post delves into the key concepts of reinforcement learning, including verifiable rewards, and explores real-world applications in areas like large language models. Gain insights into how this cutting-edge technique is driving advancements in artificial intelligence.
What is Reinforcement Learning?
The Importance of Verifiable Rewards
Real-World Applications of Reinforcement Learning with Verifiable Rewards
Conclusion
What is Reinforcement Learning?
What is Reinforcement Learning?
Reinforcement learning is a technique used to elicit thinking behavior from AI models. It involves an AI agent interacting with an environment, where the environment provides feedback to the agent in the form of rewards. The agent then learns to maximize these rewards through its actions, even if it doesn't fully understand the reasoning behind them.
The key aspect of reinforcement learning is the use of verifiable rewards. Verifiable rewards are reward signals that come from objectives that can be automatically verified, such as solving a math problem or correctly executing a computer program. This ensures that the agent is being rewarded for achieving the desired outcome, rather than finding unintended loopholes or "reward hacking" as can happen with proxy rewards.
Verifiable rewards are important because they align the agent's behavior with the true goal, are resistant to reward hacking, and produce predictable and reliable behavior. They are particularly well-suited for tasks in STEM fields, where there is often a clear definition of the correct answer.
By incorporating reinforcement learning with verifiable rewards, large language models like GPT-based models can be fine-tuned to excel at specific tasks, such as solving multiplication tables or tackling advanced logic and reasoning problems. This technique is a key driver of the "thinking" behavior exhibited by the latest AI models.
The Importance of Verifiable Rewards
The Importance of Verifiable Rewards
Verifiable rewards are incredibly important in the world of thinking models, as they are the key aspect that allows these models to scale and develop true thinking capabilities. Verifiable rewards are reward signals that come from objectives that can be automatically verified, ensuring that the reward truly represents the desired outcome.
The key benefit of verifiable rewards is that they ensure alignment with the desired behavior. Unlike proxy rewards, which can lead to unintended behaviors as seen in the boat racing game example, verifiable rewards leave no room for interpretation or loopholes. The agent either achieves the goal or it doesn't, and it is programmatically determined whether the model got something right or wrong.
Verifiable rewards are also highly resistant to reward hacking, as the result must exactly match the ground truth. This makes the model's behavior more predictable and reliable, reducing the likelihood of producing bizarre or unintended outcomes.
Furthermore, verifiable rewards are bias-free and objective, unlike reward schemes based on human preferences, which can be influenced by human biases. This allows the model to learn in a safer and more trustworthy manner.
The main areas where we see reinforcement learning with verifiable rewards being applied are in STEM-related tasks, such as solving math problems, writing computer programs, and other areas where there is a clear definition of the correct answer. Large language models, such as GPT-based models, are also benefiting from this approach, as it allows them to develop more advanced thinking capabilities.
Real-World Applications of Reinforcement Learning with Verifiable Rewards
Real-World Applications of Reinforcement Learning with Verifiable Rewards
Reinforcement learning with verifiable rewards has found numerous real-world applications, particularly in domains that involve clear definitions of inputs and outputs, such as STEM (Science, Technology, Engineering, and Mathematics) fields.
One of the primary areas where this technique is being leveraged is in the development of large language models. These GPT-based models are initially trained on vast amounts of data, and then further fine-tuned using reinforcement learning with verifiable rewards. This approach allows the models to excel at tasks with clear, verifiable solutions, such as solving multiplication tables or tackling advanced logic, reasoning, and coding challenges. By providing the models with feedback that can be objectively verified, the reinforcement learning process helps to elicit the desired "thinking" behavior, enabling the models to perform increasingly complex cognitive tasks.
Additionally, reinforcement learning with verifiable rewards has applications in areas where there is a clear definition of success or failure, such as in computer programming, where the output of a program can be directly compared to the expected result. This approach can help AI agents learn to write more reliable and efficient code, as they are rewarded for producing outputs that match the desired outcome.
Furthermore, this technique can be beneficial in scientific and engineering domains, where there are often well-defined problems with verifiable solutions. For example, in physics or chemistry, AI agents can be trained to solve complex equations or simulate experiments, with the reinforcement learning process guiding them towards the correct answers.
Overall, the use of reinforcement learning with verifiable rewards represents a significant advancement in the field of artificial intelligence, as it allows for the development of more reliable, trustworthy, and predictable AI systems that can tackle a wide range of real-world challenges.
Conclusion
Conclusion
Reinforcement learning with verifiable rewards is a powerful technique that is enabling the development of advanced AI models capable of complex reasoning and problem-solving. By providing the AI agent with clear, objectively verifiable feedback on its actions, this approach helps to ensure that the agent's behavior is aligned with the desired outcomes, and prevents the agent from exploiting loopholes or engaging in unintended behaviors.
The key advantages of this approach include:
-
Alignment with Desired Behavior: Verifiable rewards ensure that the agent is working towards a clearly defined goal, rather than optimizing for a proxy metric that may not accurately reflect the true objective.
-
Resistance to Reward Hacking: Because the rewards are based on objectively verifiable criteria, it is much more difficult for the agent to find ways to "cheat" the system and earn rewards without actually achieving the desired outcome.
-
Bias-free and Objective: Unlike reward schemes based on human preferences, verifiable rewards are determined programmatically, without the introduction of human biases or subjectivity.
-
Predictable and Reliable Behavior: The lack of ambiguity in the reward structure leads to more predictable and trustworthy behavior from the AI agent, reducing the risk of unexpected or harmful actions.
Real-world applications of reinforcement learning with verifiable rewards are particularly well-suited to domains with clear, well-defined inputs and outputs, such as STEM fields, programming, and certain language tasks. As these techniques continue to be refined and applied to more complex problems, we can expect to see increasingly sophisticated and capable AI systems that can tackle a wide range of challenging tasks.
FAQ
FAQ