Framtiden för AI-agenter: LangChain-VD avslöjar de senaste insikterna
Framtiden för AI-agenter: LangChain-VD avslöjar de senaste insikterna - Lär dig om de senaste utvecklingarna inom AI-agenter, inklusive planering, användarupplevelse och minneshantering. Upptäck hur agentramverk utvecklas för att leverera mer tillförlitliga och engagerande AI-upplevelser.
23 mars 2025
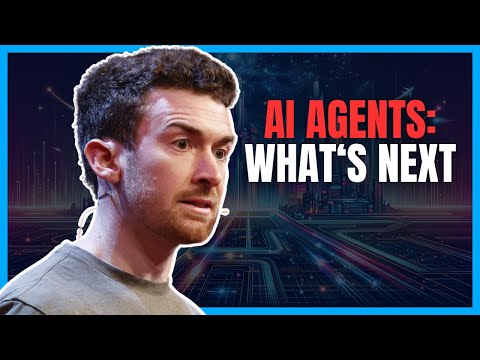
Upptäck framtiden för AI-agenter och hur de revolutionerar sättet vi interagerar med teknik. Detta blogginlägg fördjupar sig i de nyckelområden som formar framtiden för agenter, inklusive planering, användarupplevelse och minne. Få insikter från en branschledare om framsteg och utmaningar inom detta snabbt utvecklande område.
Vikten av planering för agenter
Användarupplevelsen av agentapplikationer
Minnets kraft i agenter
Slutsats
Vikten av planering för agenter
Vikten av planering för agenter
Planeringen är en avgörande aspekt av agentbaserade system, eftersom den gör det möjligt för agenter att resonera kring sina handlingar, dela upp komplexa uppgifter i delmål och säkerställa en mer tillförlitlig och sammanhängande genomförande av sina mål. Som Harrison Chase betonar, är nuvarande språkmodeller ännu inte kapabla att på ett tillförlitligt sätt utföra denna typ av planering på egen hand, och utvecklare måste ofta förlita sig på externa promptningsstrategier och kognitiva arkitekturer för att genomdriva planeringsförmågor.
En av de största utmaningarna är att språkmodeller tenderar att fungera på ett mer reaktivt, "for-loop"-liknande sätt, där de genererar ett svar, utför en åtgärd och sedan genererar nästa svar. Detta kan leda till suboptimala beslut och brist på långsiktig planering. Tekniker som tree-of-thought, reflektion och nedbrytning av delmål syftar till att åtgärda detta genom att ge modellerna förmågan att resonera kring sina handlingar, planera framåt och dela upp komplexa uppgifter.
Den långsiktiga lösningen kan dock kräva en fundamental förändring av den underliggande arkitekturen för språkmodeller, bortom de nuvarande transformerbaserade modellerna, till något som kan hantera planering och resonemang mer effektivt. Detta är ett aktivt forskningsområde, där projekt som QAR (Question-Answering Reasoning) och modeller tränade att "tänka långsamt" visar lovande resultat.
Användarupplevelsen av agentapplikationer
Användarupplevelsen av agentapplikationer
Användarupplevelsen (UX) för agentapplikationer är ett område som Harrison är särskilt entusiastisk över. Han noterar att UX:en ännu inte har "spikats" och att mänsklig inblandning fortfarande ofta är nödvändig på grund av språkmodellernas opålitlighet och risken för hallucinationer.
Harrison lyfter fram den UX som demonstrerades i Anthropic Delphi-demot som ett positivt exempel, med möjligheten att se de olika skärmarna (webbläsare, chattfönster, terminal, kod) i en vy. Han pekar också på värdet av att ha en "spola tillbaka och redigera"-funktion, vilket gör det möjligt för användarna att gå tillbaka till ett tidigare tillstånd och göra justeringar, vilket förbättrar agentens tillförlitlighet och styrförmåga.
Dessutom diskuterar Harrison vikten av "flödesdesign" - den explicita utformningen av arbetsflödet och tillståndsmaskinen som agenten verkar inom. Han föreslår att denna flödesdesign kan hjälpa till att kompensera för vissa av språkmodellernas begränsningar, genom att lägga över planeringen och beslutsfattandet på de mänskliga ingenjörerna i förväg.
Minnets kraft i agenter
Minnets kraft i agenter
Agenter är kraftfulla verktyg som går utöver bara komplexa prompter. En av de nyckelaspekter som gör agenter så kapabla är deras förmåga att utnyttja minne, både kortsiktigt och långsiktigt.
Kortsiktigt minne gör det möjligt för agenter att lära och förbättra sig under en konversation eller interaktion, bygga på tidigare steg och anpassa sitt tillvägagångssätt därefter. Detta möjliggör en mer dynamisk och anpassningsbar interaktion, där agenten kan styras och korrigeras av användaren.
Långsiktigt minne är å andra sidan avgörande för att agenter ska kunna upprätthålla och utnyttja ett företags kunskapsbas. Detta gör det möjligt för agenter att ha en djup förståelse för företaget, dess processer och relevant information, vilket gör dem mer effektiva i sina uppgifter. Hanteringen av långsiktigt minne medför dock sina egna utmaningar, såsom att avgöra vad som ska lagras, när något ska glömmas bort och hur minnet ska utvecklas i takt med att företaget förändras.
Integrationen av både kortsiktigt och långsiktigt minne i agentramverk är ett aktivt forsknings- och utvecklingsområde. När dessa förmågor fortsätter att förbättras kommer agenter att bli allt mer tillförlitliga, personliga och värdefulla i företagsmiljöer, där konsekvens och kvalitet är av största vikt.
FAQ
FAQ