AGI Is Closer Than We Think: OpenAI Researcher's Bold 3-5 Year Prediction
Explore an OpenAI researcher's bold 3-5 year prediction for the timeline of Artificial General Intelligence (AGI). Dive into the key components of general intelligence and insights on the progress in world models, system 2 thinking, and embodiment. Discover the potential convergence of robotics and large language models hinting at an exciting AGI future.
July 13, 2025
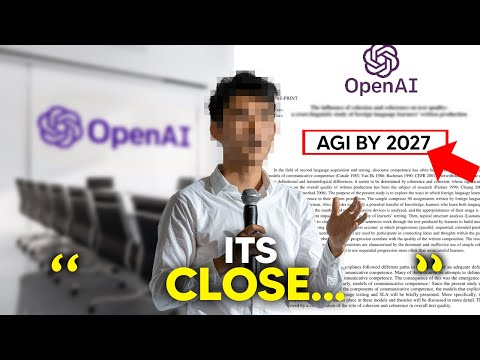
Discover the remarkable insights from an OpenAI researcher on the rapid advancements in artificial general intelligence (AGI) and how we may be closer to this milestone than you think. Explore the key components needed to build a generally intelligent agent and learn about the potential timeline for achieving AGI in the coming years.
The Key Components of a Generally Intelligent Agent
Building World Models and Improving Robustness
Skeptics, Transformers, and the Path to AGI
The Importance of System 2 Thinking
Embodiment and the Convergence of Robotics and Language Models
The Researcher's Optimistic Timelines for AGI
The Key Components of a Generally Intelligent Agent
The Key Components of a Generally Intelligent Agent
A generally intelligent entity requires a synthesis of three key components:
-
A way of interacting with and observing a complex environment: This typically means embodiment - the ability to perceive and interact with the natural world using various sensory inputs like touch, smell, sight, etc. This allows the entity to build a robust world model covering the environment.
-
A mechanism for performing deep introspection on arbitrary topics: This is the capacity for reasoning, or "slow thinking" (system 2 thinking), where the entity can think deeply about problems and devise plans to solve them.
-
A world model covering the environment: This is the mechanism that allows the entity to perform quick inferences with reasonable accuracy, akin to human "intuition" or "fast thinking" (system 1 thinking).
With these three components, the entity can be "seeded" with objectives, and use its system 2 thinking in conjunction with its world model to ideate ways to optimize for those objectives. It can then take actions, observe the results, and update its world model accordingly. This cycle can be repeated over long periods, allowing the entity to coherently execute and optimize for any given objective.
The key is that the entity does not necessarily need the capacity to achieve arbitrary objectives, but rather the adaptability and coherence to continuously act towards a single objective over time. This is what defines a truly capable, generally intelligent system.
Building World Models and Improving Robustness
Building World Models and Improving Robustness
We're already building world models with autoregressive Transformers, the same architecture that we've been using recently, particularly of the Omni model variety. How robust they are is up for debate, with issues like hallucinations and other problems. However, the good news is that in the author's experience, scale improves robustness.
Humanity is currently pouring capital into scaling autoregressive models. Microsoft is pouring a lot of capital into Project Stargate in conjunction with OpenAI, and Sam Altman is seeking $7 trillion in capital (though this is likely a clickbait headline). As long as the scale keeps increasing, the robustness of these world models should improve.
The author suspects that the world models we have right now are sufficient to build a generally intelligent agent. He also suspects that robustness can be further improved via the interaction of system 2 thinking (deep, deliberate reasoning) and observing the real world - a paradigm that hasn't really been seen in AI yet, but is a key mechanism for improving robustness.
While LLM skeptics like Yan LeCun say we haven't yet achieved the intelligence of a cat, the author argues that LLMs could learn that knowledge given the ability to self-improve. He believes this is doable with Transformers and the right ingredients.
The author is quite confident that it is possible to achieve system 2 thinking within the Transformer paradigm with the technology and compute available right now. He suspects we'll be able to build a mechanism for effective system 2 thinking within 2-3 years, which would be a key component of building a generally intelligent agent.
Regarding embodiment, the author is also quite optimistic about near-term advancements. He sees a convergence happening between the fields of robotics and large language models, which could lead to impressive demonstrations in the next 1-2 years.
In summary, the author believes we've solved building world models, have 2-3 years to solve system 2 thinking, and 1-2 years to solve embodiment. Once these key ingredients are in place, integrating them together into the cycling algorithm he described could take another 1-2 years. His current estimate for AGI is 3-5 years, leaning towards 3 years for something resembling a generally intelligent embodied agent.
Skeptics, Transformers, and the Path to AGI
Skeptics, Transformers, and the Path to AGI
While LLM skeptics like Yan LeCun say we haven't yet achieved the intelligence of a cat, this is the point they are missing. Yes, LLMs still lack some basic knowledge that every cat has, but they could learn that knowledge given the ability to self-improve. Such self-improvement is doable with Transformers and the right ingredients.
There is not a well-known way to achieve "system 2 thinking" - the long-term reasoning that AI systems need to effectively achieve goals in the real world. However, the author is quite confident that it is possible within the Transformer paradigm with the technology and compute available. He expects to see significant progress on this in the next 2-3 years.
Similarly, the author is optimistic about near-term advancements in embodiment. There is a convergence happening between the fields of robotics and LLMs, as seen in impressive demos like the recent Digit robot. Large language models can map arbitrary sensor inputs into commands for sophisticated robotic systems.
The author has been testing GPT-4's knowledge of the physical world by interacting with it through a smartphone camera. While not perfect, it is surprisingly capable, and the author suspects we'll see some really impressive progress in the next 1-2 years in deploying systems that can take coherent strings of action in the environment and observe the results.
In summary, the author believes we've solved the problem of building world models, and with 2-3 years of progress on system 2 thinking and 1-2 years on embodiment, we can integrate these capabilities into a cycling algorithm for a generally intelligent, embodied agent. His current estimate for AGI is 3-5 years, with the first iteration looking a lot like AGI in 3 years, followed by further refinement to convince even the skeptics.
The Importance of System 2 Thinking
The Importance of System 2 Thinking
The author emphasizes the critical role of "system 2 thinking" in building generally intelligent agents. System 2 thinking refers to the mechanism for performing deep introspection and reasoning on arbitrary topics, as opposed to the more intuitive "system 1 thinking" that relies on fast, automatic responses.
The author argues that for an agent to be generally intelligent, it needs to have a way of interacting with and observing the environment (embodiment), a robust world model covering the environment (intuition/system 1 thinking), and a mechanism for deep introspection and reasoning (system 2 thinking).
Specifically, the author states that with the world models currently available, he suspects they are sufficient to build a generally intelligent agent. However, the key missing piece is the system 2 thinking capability. The author is confident that it is possible to achieve effective system 2 thinking within the transformer paradigm, given the current technology and compute available.
The author estimates that developing a robust system 2 thinking mechanism will take 2-3 years. Combined with 1-2 years for improving embodiment capabilities, the author predicts that we could see the emergence of a generally intelligent, embodied agent within 3-5 years. This would represent a major milestone on the path towards AGI.
The author emphasizes the importance of system 2 thinking, noting that it is a critical component that allows agents to coherently execute cycles of planning, action, and observation over long time periods to optimize for their objectives. Improving this capability is seen as a key focus area for making significant progress towards AGI.
Embodiment and the Convergence of Robotics and Language Models
Embodiment and the Convergence of Robotics and Language Models
The author expresses optimism about the near-term advancements in the embodiment of AI systems. He notes a convergence happening between the fields of robotics and large language models (LLMs).
The author highlights recent impressive demonstrations, such as the Figure robot that combined the knowledge of GPT-4 with fluid physical movements. He also mentions the recently released Unitary H1, an AI agent avatar that resembles a Boston Dynamics robot.
The author explains that large omnimodal models can map arbitrary sensory inputs into commands that can be sent to sophisticated robotic systems. This allows for the deployment of systems that can perform coherent strings of actions in the environment and observe and understand the results.
The author has been spending time testing GPT-4's knowledge of the physical world by interacting with it through a smartphone camera. While not perfect, he finds it surprisingly capable, and suspects we will see impressive progress in the next 1-2 years in this area.
The author summarizes that we have essentially solved the problem of building world models, and have 2-3 years until we can achieve effective system 2 thinking (long-term reasoning). Concurrently, he expects 1-2 years of progress on embodiment. Once these key ingredients are in place, integrating them into the cycling algorithm described earlier will take another 1-2 years.
Overall, the author's current estimate for achieving AGI is 3-5 years, leaning towards 3 years for something resembling a generally intelligent embodied agent, which he would personally consider an AGI. However, he acknowledges it may take a few more years to convince more skeptical figures like Gary Marcus.
The Researcher's Optimistic Timelines for AGI
The Researcher's Optimistic Timelines for AGI
The researcher believes that the key components for building a generally intelligent agent are already within reach. He outlines a three-part definition of general intelligence:
- A way of interacting with and observing a complex environment, typically through embodiment and the ability to perceive and interact with the natural world.
- A robust world model covering the environment, allowing for quick and accurate inferences - what humans refer to as "intuition" or "system 1 thinking".
- A mechanism for performing deep introspection and reasoning on arbitrary topics - "system 2 thinking" or deliberate, conscious thought.
The researcher argues that with these three components, it is possible to build a generally intelligent agent that can coherently execute a cycle of planning, acting, observing, and updating its world model to optimize for given objectives.
He believes that the world models built with current large language models are already sufficient to construct such a generally intelligent agent. The key remaining challenges are:
- Developing effective "system 2 thinking" capabilities within the transformer paradigm. The researcher is confident this can be achieved in the next 2-3 years.
- Integrating embodied interaction with the physical world. He expects significant progress in this area in the next 1-2 years.
By combining these advancements, the researcher estimates that we could see the emergence of an "embodied, generally intelligent agent" that he would personally call an AGI within 3-5 years. He leans towards the 3-year timeline, though notes it may take additional time to convince more skeptical figures in the field.
Overall, the researcher presents an optimistic view of the path towards AGI, centered around developing robust world models, deliberative reasoning, and physical embodiment within the next 3-5 years. This timeline aligns with other prominent predictions, such as Anthropic co-founder Dario Amodei's estimate of AGI by 2027.
FAQ
FAQ